Across all medical disciplines, artificial intelligence and machine learning will transform medicine beyond most people’s imagination. Algorithms that help evaluate radiological images are just the beginning. AI could become an indispensable tool in all branches of medicine.
Download your PDF here.
Photos: Gremlin/Getty Images, Anna Schroll, Philip Frowein
From virtual assistants in the living room to intelligent investment algorithms and from software-based traffic control to autonomous driving – there is no escaping artificial intelligence (AI) and machine learning. The topic is also currently being addressed at the political level. China presented its Next Generation Artificial Intelligence Development Plan as far back as July 2017. In March 2018, French President Emmanuel Macron announced that his government would invest a total of €1.5 billion in AI by 2022. In April 2018, the British government launched its £1 billion AI Sector Deal policy paper. In May, it was Sweden’s turn. The German government unveiled its AI strategy at the end of 2018. At the European level, the European Commission formulated a European AI Strategy in April 2018, setting out the draft European AI ethics guidelines a year later.
Practical uses for AI thanks to increased computing power
The advance of AI can be seen in medicine, as well. From prevention and screening to diagnosis, therapy, and disease management, innovative companies and technology-oriented medical institutions have been developing, testing, and – increasingly – implementing intelligent algorithms in virtually every branch of medical care. At the same time, regulators are turning their attention to the topic. In February 2019, the U.S. Food and Drug Administration (FDA) released a discussion paper regarding the licensing of AI applications for medicine. It is not concerned with ‘simple’ applications that are trained and re-trained during updates – such solutions already exist and have regulatory approval. Rather, the new FDA initiative concerns more sophisticated AI systems that learn in real time and are constantly changing their algorithms, requiring them to be regulated differently from traditional software solutions.
The distinction between ‘static’ and ‘dynamic’ AI, which the FDA is now also addressing, shows that artificial intelligence is a very broad, vaguely defined term. Today, when people talk about AI, they usually mean deep learning, a special form of machine learning using artificial neural networks. It has become known through the Google algorithm which succeeded in beating the world Go champion. But in fact, neural networks have existed for decades. “Computing power is what has changed most. The increase in power has allowed for algorithms, which have been around for a long time, to be put to practical use,” says Bram Stieltjes, MD, Head of Research Coordination at the Radiology & Nuclear Medicine Clinic at University Hospital Basel, Switzerland. Graphics cards – essential tools for training neural networks – have also become much more powerful.
“Mathematical revolution in radiology”
This training of neural networks – often involving tens of thousands of datasets – has made headlines, especially in radiology where algorithms now perform better than radiologists on certain questions. Professor Stefan Schönberg, Chairman of the Department of Clinical Radiology and Nuclear Medicine at the University Medical Centre Mannheim, Germany, speaks of a “mathematical revolution in radiology”. Analyzing images is one thing, he says. But in an age of radiomics, algorithms sift through multidimensional data sets using high resolution that investigate data sets down to single voxels. “It’s not at all clear whether we are looking at images or at purely statistical parameters,” Schönberg says.
It’s not at all clear whether we are looking at images or at purely statistical parameters.
The fear, as it is sometimes spread by the media, that algorithms could replace radiologists is not shared by experts: “Most radiologists don’t see AI as a threat but as something beneficial to the field,” says Professor Michael Forsting from the Institute of Diagnostic and Interventional Radiology and Neuroradiology at Essen University Hospital, Germany. Algorithms can handle the repetitive, time-consuming activities and thereby lighten the radiologists’ everyday workload, according to Forsting. “For example, here in Essen we have automated the counting of multiple sclerosis lesions in the brain. We also use algorithms to determine bone age, and in the early detection of strokes.”
Most radiologists don’t see AI as a threat but as something beneficial to the field.
AI to make inroads into labs
In other diagnostic disciplines, self-learning algorithms could lead to far-reaching change in the next few years. Right at the forefront is pathology, which produces enormous datasets that have not yet been fully evaluated. “The first step will definitely be an increase in efficiency thanks to AI,” underlines Professor Frederick Klauschen from the Institute of Pathology at Charité in Berlin, Germany, at an event of the Federal Association of German Pathologists. Pathologists think it likely that algorithms will quite soon, for instance, take over the task of counting cell nuclei. This would free them and their colleagues up for more complex tasks.
The first step will definitely be an increase in efficiency thanks to AI.
In the medium term, Klauschen can also imagine algorithms being used for quite different evaluations, including analyses that are difficult without AI. For instance, there are indications that self-learning algorithms are better than humans at recognizing complex biomarker patterns in cancer patients. These patterns could then be used in precision medicine instead of single parameters to predict which patients will respond to immunotherapies.
With regard to biomarkers, AI algorithms are also likely to trigger some developments in laboratory medicine – the third major diagnostic field aside from pathology and radiology. In a Siemens Healthineers survey of 200 clinical laboratory executives, seven out of ten respondents said AI would move into in vitro diagnostics (IVD) over the next four years. As many as nine out of ten were convinced that AI would have a significant long-term impact on healthcare. Every second respondent had already been using AI applications in laboratory medicine.[1]
Will diagnostic specialists become redundant?
In laboratory work, there is an interest in algorithms that support operational processes. For example, in cross-lab monitoring of diagnostic systems, AI can detect problems before failures occur, allowing for proactive maintenance schedules. On the clinical side, algorithms are suited to diagnostic decision-making in laboratory medicine and also, similar to pathology, to predictive analytics based on complex biomarker patterns.
One particularly promising use is the holistic analysis of diagnostic information, in which algorithms collate data from the laboratory, electronic patient record, imaging, and sometimes pathology. Bram Stieltjes of University Hospital Basel sees this AI-supported ‘interdisciplinarity’ as one of the ways in which algorithms directly impact clinical practice in the diagnostic fields: “It’s possible that the roles of radiologist, pathologist, and laboratory physician will cease to be separate in the future. Perhaps we will become total integrators of diagnostic information, working together more closely in integrated diagnostic departments to bring together all the pieces of the diagnostic puzzle as quickly as possible.”
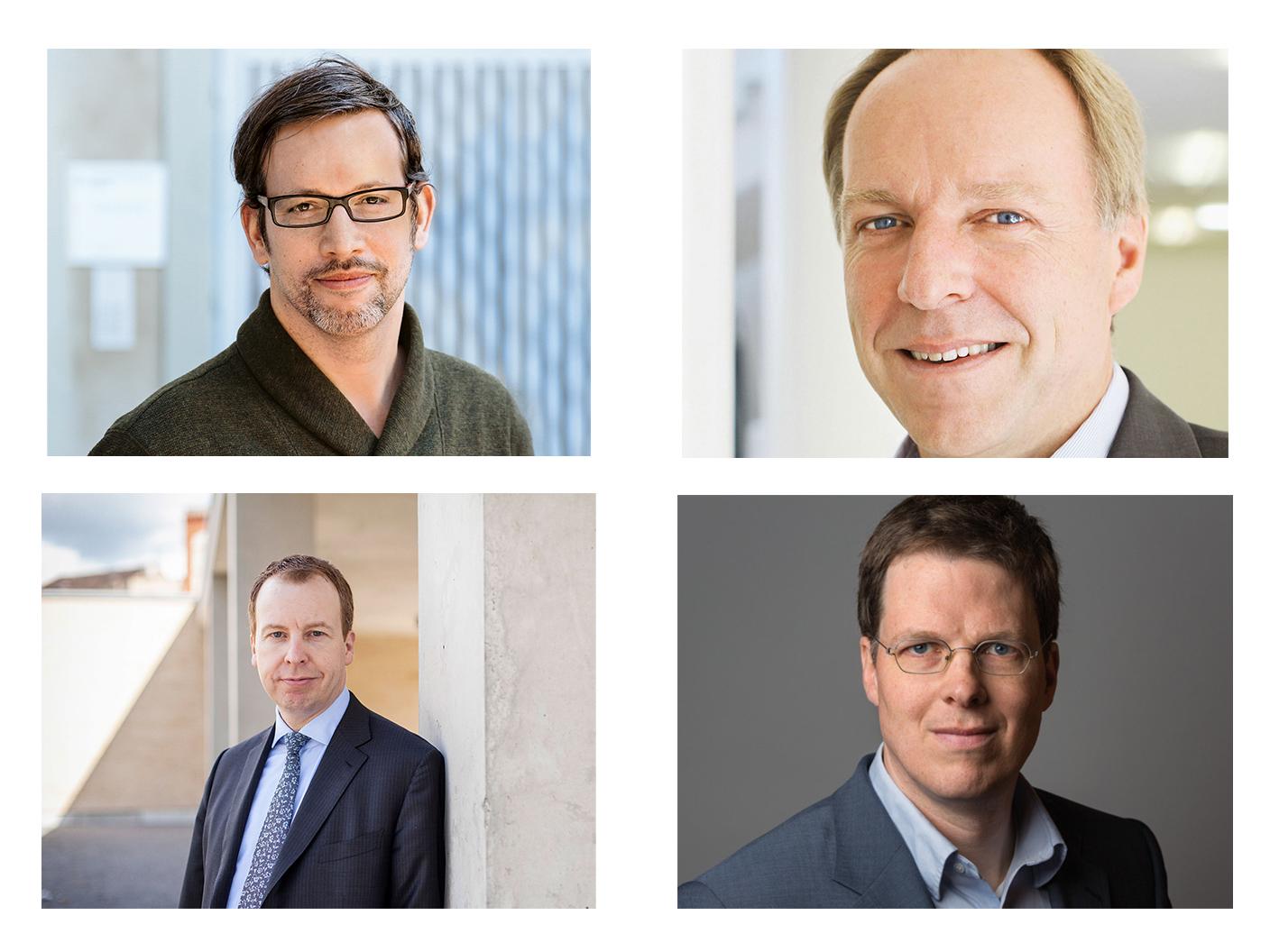
AI and narrative-based medicine
Radiology, pathology, and laboratory medicine – these are all very technical fields that are already highly digitized in many areas. It comes as no surprise that this is where a lot of discussions about medical AI are taking place. Michael Forsting, who is responsible for the annual Emerging Technologies in Medicine (ETIM) congress – which addresses medical AI applications far beyond radiology – is convinced this is a passing phenomenon: “In the long run, the technical disciplines will change much less than narrative-based and clinical medicine.”
Why is that? “Simply because most mistakes happen in non-technical areas, and AI can reduce errors,” says Forsting. As an example, the radiologist cites algorithm-based applications that use voice, facial expression, and posture to make a tentative diagnosis of depression. These algorithms are increasingly being tested in clinical trials. They could be very useful, for instance in situations where a doctor who is not a psychiatrist sees a patient who is supposedly suffering from a purely physical ailment.
Geneticists and bioinformaticians from the U.S. and Germany reported on a rather different use in the journal Nature Medicine at the start of 2019. The scientists have developed a network of algorithms called DeepGestalt, which has been trained to detect rare genetic diseases through facial photography. After training on 17,000 images of patients with over 200 different genetic syndromes, it analyzed a total of 502 facial photographs of a typical patient group in human genetic consultations. In nine out of ten patients, the correct diagnosis was among the first ten suggestions.[2] DeepGestalt could be of enormous help, especially in regions where there are no rare hereditary disease specialists. “AI can bring about huge improvements in narrative-based and clinical medicine,” Forsting is convinced.
About the Author
Philipp Grätzel von Grätz is a Berlin-based medical doctor turned independent writer. His focus is on biomedicine, medical technology, health IT, and health policy.