The broader implementation of artificial intelligence (AI) will be based on versatile systems that can be seamlessly integrated into existing workflows and IT architectures. Assessing multiple anatomical structures and organs on a chest CT more quickly and precisely would be one strategy to make AI support a self-evident aspect of image interpretation.
Download the whitepaper here.
A crucial prerequisite for advancing the implementation of AI and fully exploiting its benefits is the availability of easy-to-use, comprehensive solutions for clinical routine In particular, compatibility with existing picture archiving and communication systems (PACS) is key to the successful use of AI in healthcare organizations. “Ultimately, the driver of clinical adoption may reside in the implementation and availability of AI applications integrated into the PACS system at the reading station,” confirms a technology white paper from the Canadian Association of Radiologists [1]. In other words: AI should not reinvent workflows, but instead improve and accelerate what radiologists do every day in as many different ways as possible.
In general, experts assume that completely independent diagnostic algorithms will find their way into radiological routines only in the medium to long term and that AI will, in the near future, rather serve to accelerate workflows and facilitate image interpretation [2].
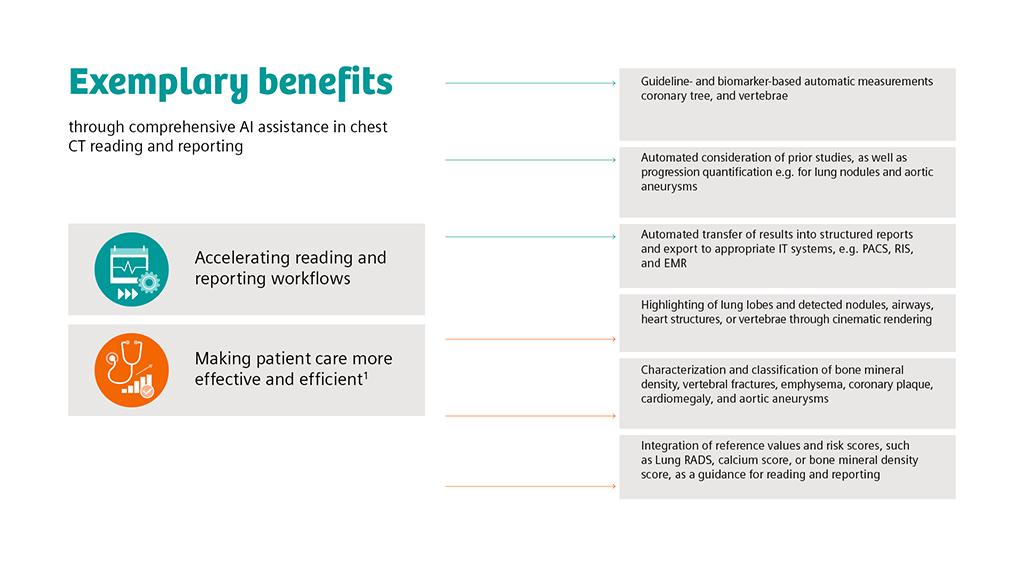
Chest Imaging Shows Tangible Benefits
An exemplary case for AI-supported multifunctional image analysis is chest imaging, which already is one of the most important radiological fields of work. Lung cancer screening with low-dose CT in particular could further increase the need for fast and reliable image interpretation in many countries worldwide in the coming years.
The mere ability to automatically locate suspicious lesions using AI-powered lung segmentation [3] and to measure their size in 2D and 3D could save an enormous amount of time. Equally promising are algorithms for determining airway obstructions in COPD and the extent of emphysema (Das et al. 2018) or for quantifying the severity of pulmonary fibrosis on CT scans [4]. Last but not least, AI-supported 3D visualizations such as cinematic renderings can simplify the reading process and make it more intuitive [5].
An envisioned advantage of an organ-spanning AI system is that, for example, cardiopulmonary diseases are easier to assess and incidental findings are less often missed. Due to their high spatial and temporal resolution, modern scanners enable comprehensive cardiothoracic evaluations even on non-ECG-synchronized, non-contrast-enhanced thoracic CTs [6]. Indeed, up to two-thirds of incidental cardiac findings detectable on a noncardiac CT, such as coronary calcifications or aortic dilatation, remain unmentioned in the radiological report [7, 8].The same is true for thoracic bone tumors or metastases, which are by no means rare or unexpected findings on chest CTs but still tend to slip through the diagnostic net, with potentially serious clinical consequences [9].
For metastasis detection in particular, it is desirable to have assisting AI platforms available in the future to evaluate not only images of individual body areas such as the chest, but also whole-body scans. For example, in advanced stages of breast or prostate cancer, metastases typically occur in the skeleton, or in the lungs, liver, or brain. Here, further developed, integrated AI systems may significantly improve whole-body evaluation in the coming years.
Continuous Improvements
Artificial intelligence is a learning technology. New architectures of artificial neural networks have made possible remarkable progress in image analysis in recent years and will most probably continue to do so in the future. Also, it is in the nature of intelligent algorithms themselves that they learn by processing large amounts of data and adjust and optimize their internal parameters. This optimization process is key, in particular when AI applications are used with different scanners or imaging protocols, or in different patient populations. It is therefore obvious that AI systems need regular, well-planned updates to take full advantage of the technology. For AI-supported image interpretation, this means that solutions that are already tangible and feasible today are poised for expansion and improvement in the future. Cloud-based infrastructures and user feedback will allow algorithms to be adapted at a fast pace, and new applications to be integrated into AI systems.