Will self-learning algorithms take the place of doctors? Probably not, but a medical sector that does not leverage the strengths of artificial intelligence could soon be seen as irresponsible.
Photos: Markus Zucker
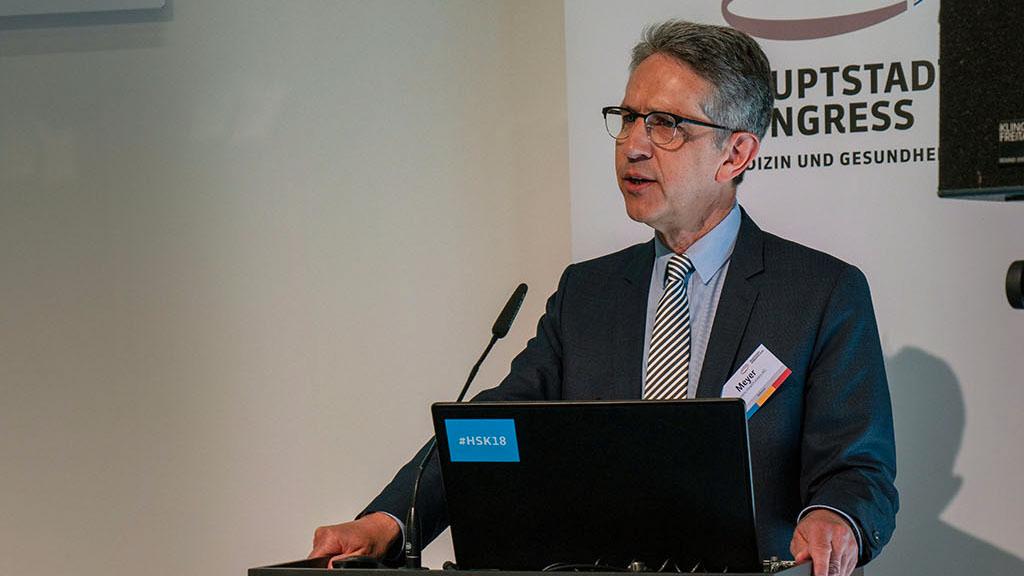
There are already obituaries being written on the age of artificial intelligence (AI) in medicine. Some projects launched with a major PR push have failed their clinical trial by fire – in oncology for instance. “Is AI just the next big thing? Are we building stadiums that will remain empty?” With these provocative questions, Michael Meyer, Head of Strategy & Business Development Germany and Head of Government Affairs & Politics CEMEA at Siemens Healthineers, opened a discussion panel on artificial intelligence in medicine. It quickly became clear, of course, that there can be no question of empty stadiums. At most it is a case of the spectators not flooding in quite as fast as some had hoped.
AI in radiology: the fourth revolution
The stands are already well filled in radiology where diagnostic tools that use AI are slowly but surely being included in patient care. The President of the German Society of Radiology (DRG), Stefan Schönberg of the Department of Clinical Radiology and Nuclear Medicine, University Medical Center Mannheim, was convinced that the use of self-learning algorithms will be the fourth, ‘mathematical’ revolution in modern radiology following the introduction of contrast medium, the technical optimization of imaging to increase speed and lower dosage, and the introduction of molecular tracers.
Schönberg stressed that the success of self-learning algorithms in medicine depended on high-quality data that could be used to train the algorithms: “That was completely overlooked in some previous projects.” For him, radiology has an obligation to provide quality-assured annotated image datasets in order to fully exploit the digital opportunities for patients. “The DRG is in the process of setting up a national, quality-assured radiomics platform to collect the necessary data. That will make a big difference, but we have to ensure the next generation is mathematically trained,” he said.
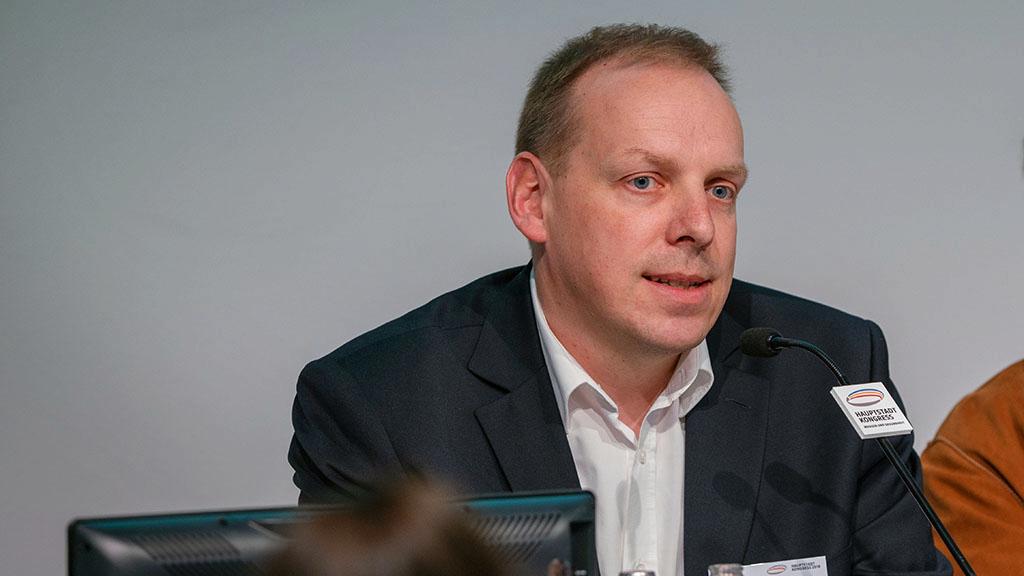
Software to calculate the ASPECTS score
Thomas Friese, Vice President Digital Ecosystem Platform at Siemens Healthineers, also underlined the importance of large datasets for the further development of AI in radiology: “We need inter-institutional, maybe even international datasets, to be able to train the algorithms.” Friese also recalled that for all the attention currently being devoted to adaptive algorithms, it is often forgotten that AI and machine learning methodologies are already widely established in radiology software products. The more recent deep learning techniques contribute not least to making programs – for instance, those that automatically recognize certain structures – even more powerful.
The assumption that use of AI applications in radiology is still a long way off, was also rejected by Michael Forsting, Director of the Institute of Diagnostic and Interventional Radiology and Neuroradiology and CIO at Essen University Hospital. At his own facility, for example, an adaptive algorithm that calculates the ASPECTS score on the basis of CT data is already used during CT scans of patients in the early stages following a stroke. The score allows a prognosis to be made for these patients. The manual calculation of the score is time-consuming and largely depends on the radiologist. The AI-based evaluation therefore has clear advantages.
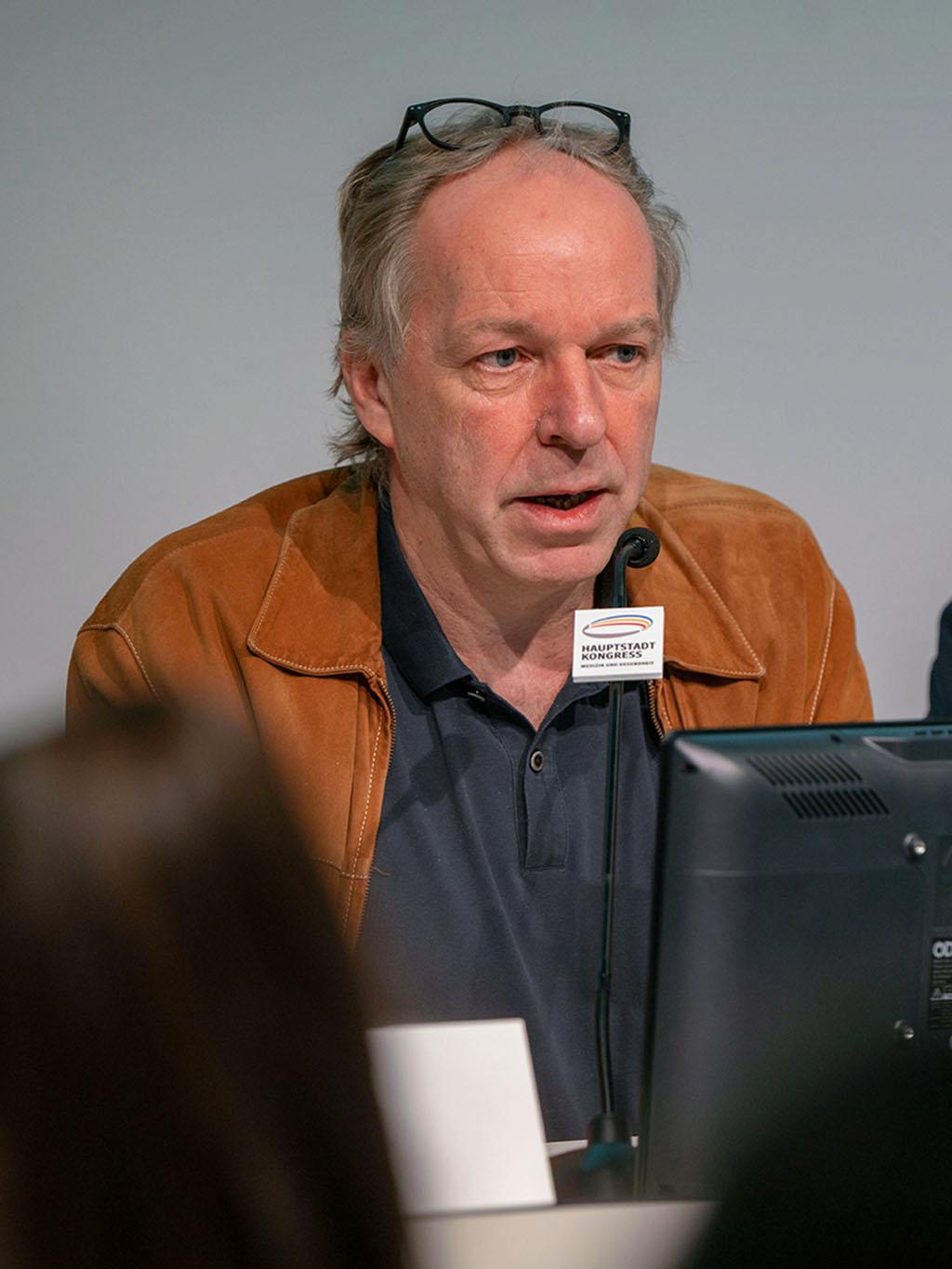
Cancer screening by Dr Algorithm
Forsting sees an evolutionary process taking place within radiology towards more and more AI applications in different situations. A particularly welcome field of application in the relatively near future will be the early detection of cancer. In this area there are already large quality-assured datasets which can be used to train algorithms very effectively: “In China mammography screenings are never done by a radiologist. It is the same with lung cancer screening; no one seriously believes any more that this will be done by radiologists.” Oncological follow-up is another area that is perfectly suited to AI systems, he said. These examinations are relatively tedious from a radiological point of view, according to Forsting. He is therefore convinced that artificial intelligence will make radiology as a subject more appealing.
Neither does Forsting foresee radiology having to deal with a wave of liability claims if software tools begin to create results. Doctors trust the results of complex laboratory automation because of the very strict quality assurance that is recognized by case law. Radiological AI systems could also follow this model. It is much more likely that doctors will have liability problems in future if they do not make use of AI tools and overlook a finding that the software would have picked up.
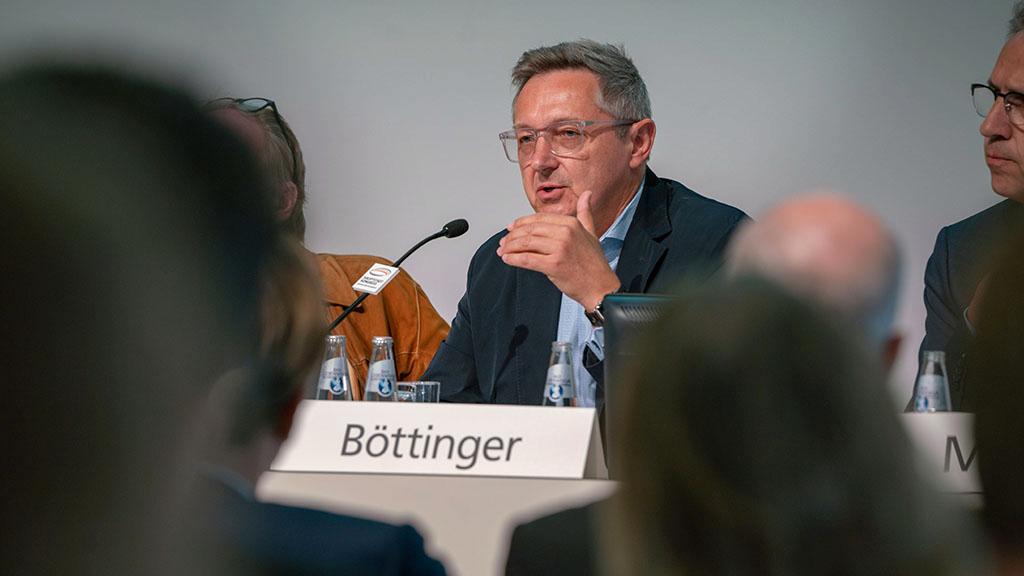
Is Germany failing to keep pace?
Erwin Böttinger, Chair of Digital Health and Personalized Medicine at the Hasso Plattner Institute, pointed out that the discussion surrounding self-learning algorithms in medicine is about much more than optimizing medical diagnostic devices. Substantial progress in the area of disease prevention and prediction is to be expected when self-learning algorithms are used to analyze complex health matters that include not just image data, but also clinical data from across the care continuum and consumer-generated data.
However, implementing this is a huge problem, especially in Germany, says Böttinger: “Outside the diagnostic fields, we do not have the necessary data context in Germany. Due to the segregation of care sectors, we lack the overview. For German companies this is a major competitive disadvantage.” Böttinger sees promising applications for AI algorithms in the area of comprehensive, broad-based health data analysis, notably in the relatively early prediction of serious health events such as psychiatric crises or acute myocardial infarction and strokes. If algorithms are successful in helping detect periods of increased risk, this would give rise to entirely new approaches to targeted preventive therapies, he believes.
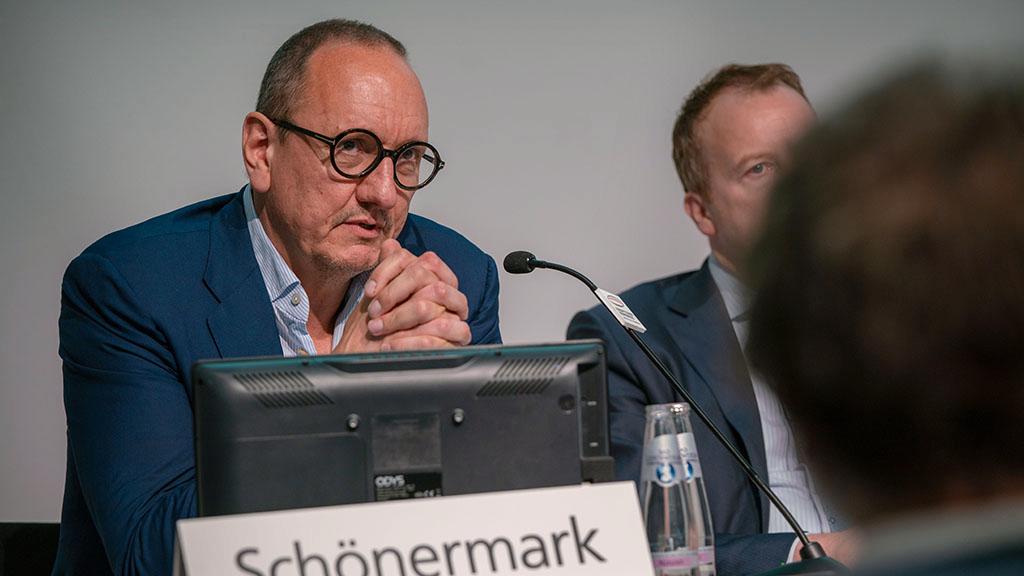
Business models are often still unclear
Another problem for AI use in healthcare, apart from the provision of suitable large datasets for the training of the algorithms, is its financing, said Matthias Schönermark of SKC management consultants for healthcare. In this opinion, the business models for the use of self-learning algorithms are in many instances completely unclear. Above all, there needs to be a discussion about incentive schemes to enable new technologies such as AI applications to be introduced on a broad scale. “Our hypothesis is that in artificial intelligence only those business models will succeed which have a perceptible and objectifiable benefit for the patient,” said Schönermark.
About the Author
Philipp Grätzel von Grätz works as a freelance journalist and editor in Berlin covering medical and technology topics.