Artificial intelligence (AI) promises to augment workflows in radiology in many ways, by providing supportive tools particularly for highly standardized and repetitive tasks, starting from the identification and delineation of anatomical structures and organs and the corresponding extraction of quantitative parameters.
The concept, in fact, is not entirely new. Tools based on machine learning algorithms applied to medical
images have been in wide clinical use for many years, as in MRI exam planning support provided by Siemens’ Dot Engines. What is new is that the latest technical advances promise to improve the performance of such tools to a level that will extend their usefulness to many more use cases than could be covered previously.
Among the many use cases of AI related to MR imaging, which range from exam planning to image reconstruction, to image interpretation, and to clinical decision support, let us now focus specifically on image analysis and interpretation and how AI tools may help in the process at different levels (Fig. 1). Going from an image series as the input to the radiology report as the result involves a number of steps. The first step is the assessment of the quality of the image. Next, there are the localization and delineation of anatomical structures in the image.
Such results may already be highly useful in their own right because they relieve clinical caregivers of tedious work, e.g., in automated scan planning, or organ contouring for radiotherapy. Then the measurement and quantification of the morphological structures that were identified may follow, as in organ volumetry, which in fact is often only feasible in routine reading if supported by automation.
Yet another step may identify abnormalities in the image, followed by an assessment of their clinical significance. It is here that we cross into the territory of computer-aided detection (CADe) and computer-aided diagnosis (CADx).
Then there is the step of compiling the findings and results for a clinical report. Typically, this is the highest level of abstraction that is achievable based on information from images alone; in many cases the report, and certainly the recommendations for clinical follow-up, will require additional input beyond imaging, e.g., from patient anamnesis and laboratory exams.
Let us turn now to how AI tools will become available to the radiologist. It is our conviction at Siemens
Healthineers that the success of AI in clinical practice will hinge not only on the stand-alone performance and
robustness of the algorithms, but in equal measure on their seamless integration into the radiology workflow.
One example of our vision is the AI-Rad Companion2 as our platform for inserting AI into routine, PACS-based reading. The first application on this platform will be a comprehensive package of visualizations, evaluations, and quantifications for thorax CT3. Based on our teamplay infrastructure, results will be automatically generated as soon as the exam is complete, and added to the case as extra DICOM image series. In this way, they are readily accessible, along with the original images, in any viewer in which the reader may choose to open the case. In the chest CT example, the analyses cover the lung3, the aorta3,
the heart3, and the spine4, so as to address not only the organ or organs that may have been the original focus for prescribing the exam, but other anatomies that are visible on the image as well.
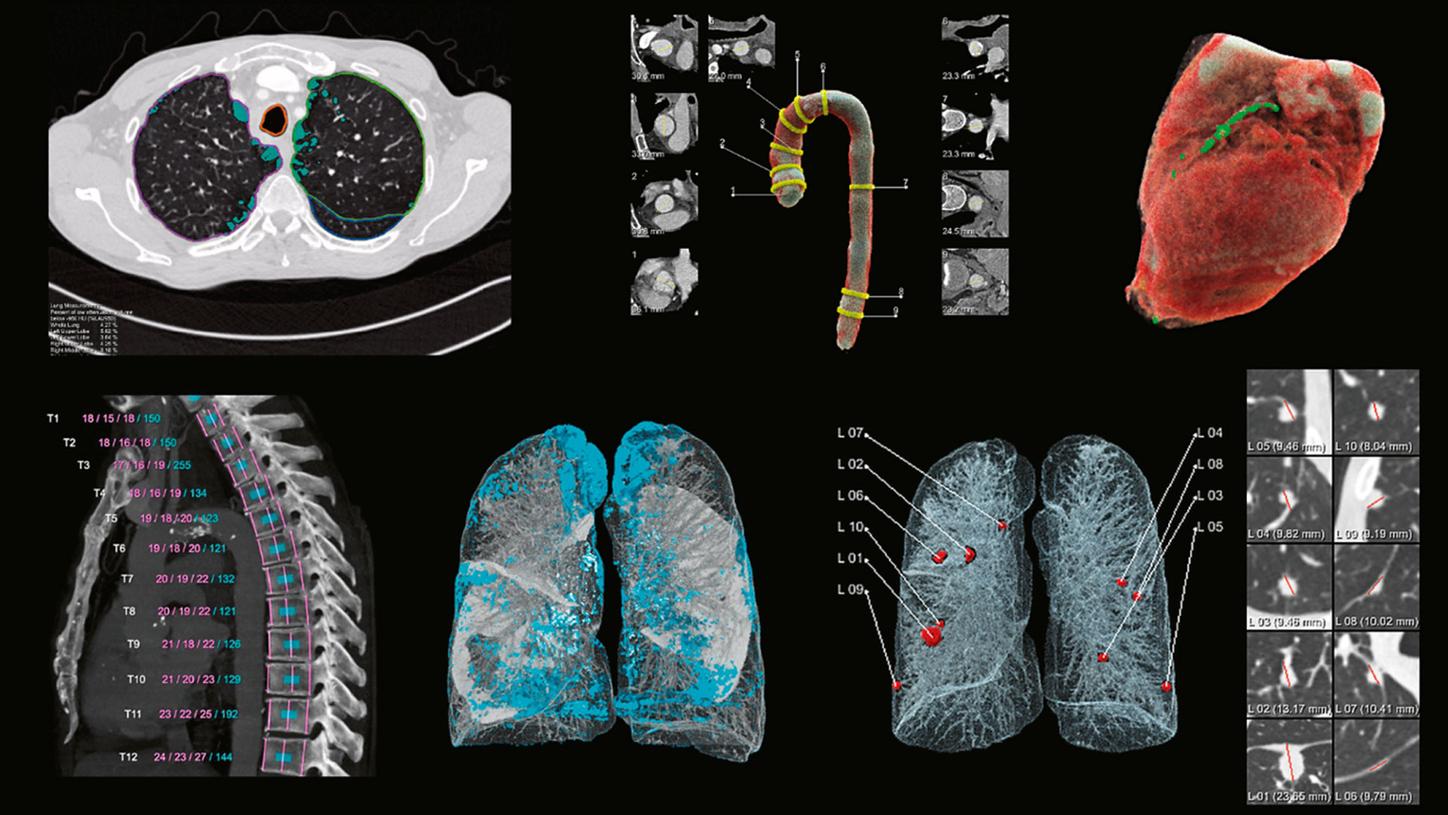
Figure 2 shows examples of the output. For the lung, evaluations comprise the detection of suspicious lung nodules including measurements of their size, as well as the visualization and quantification of volumes of low X-ray attenuation within the lung, both for the lung as a whole and for each lung lobe separately.
For the heart, a visualization and quantification of the volume of the heart and in particular of coronary calcifications is prepared. For the aorta, a segmentation is performed and visualized, along with measurements of the diameters at different positions, and for the spine4, the vertebral bodies are automatically segmented, visualized, labeled, and measured, both in height and in bone density. All of these evaluations are designed to be robust to the input variability presented by different CT scanners and acquisition protocols, and to work with and without the use of contrast agents.
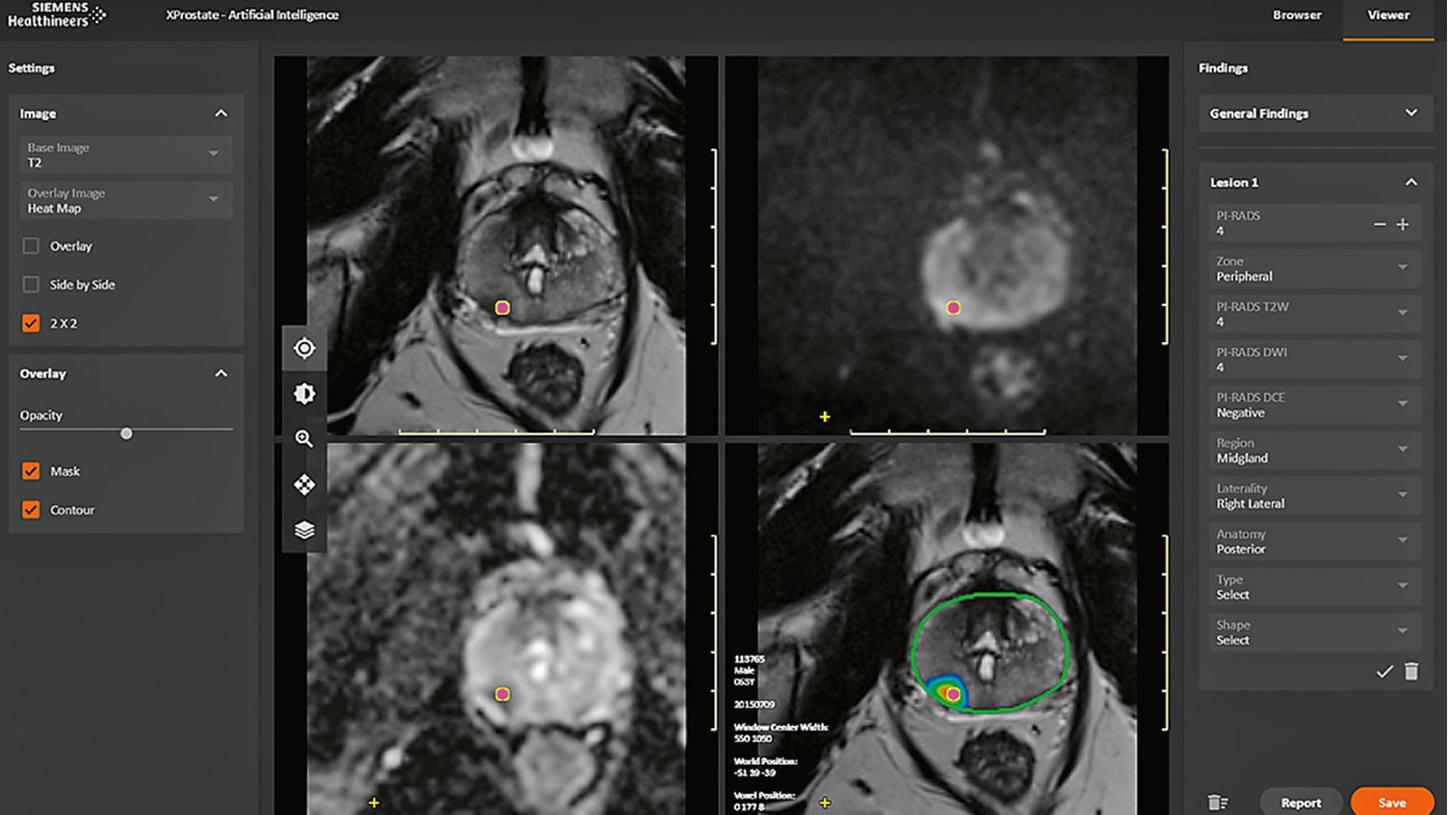
It is worth noting that chest CT imaging is just the first area for which we plan to bring to the market AI functionalities that are designed to save time and support consistent quality in the reading process. For a glimpse of our vision of the future, let us discuss another application, currently work in progress, that targets the AI analysis of multiparametric prostate MRI and is intended to cover all the analysis steps described above for image reading up to the prepopulation of a structured report. Again, the aim is to save time and support consistent quality. Figure 3 shows a screenshot of a minimal user interface geared specifically to prostate MRI reading. In this prototype, AI algorithms provide an automatic segmentation of the overall prostate and of the peripheral zone of the prostate, a map of lesions identified as suspicious, and a proposal for their PI-RADS classification, for the reader to check and edit if necessary before the results are compiled in a structured report.
There are plenty of further application scenarios involving AI beyond those presented here, such as in more efficient image reconstruction, in patient triage, and in clinical decision support. What they all have in common is a supporting role, often in well-defined, repetitive tasks. Even though they will be powerful instruments in some rather well-standardized contexts, it is to be expected that these applications will become just a new set of tools in the hands of the radiologist, assisting but not replacing the human reader. In fact, their use and usefulness may become so ubiquitous within a few years that, just as with chess programs, we will no longer even call them “AI”.
About the Author
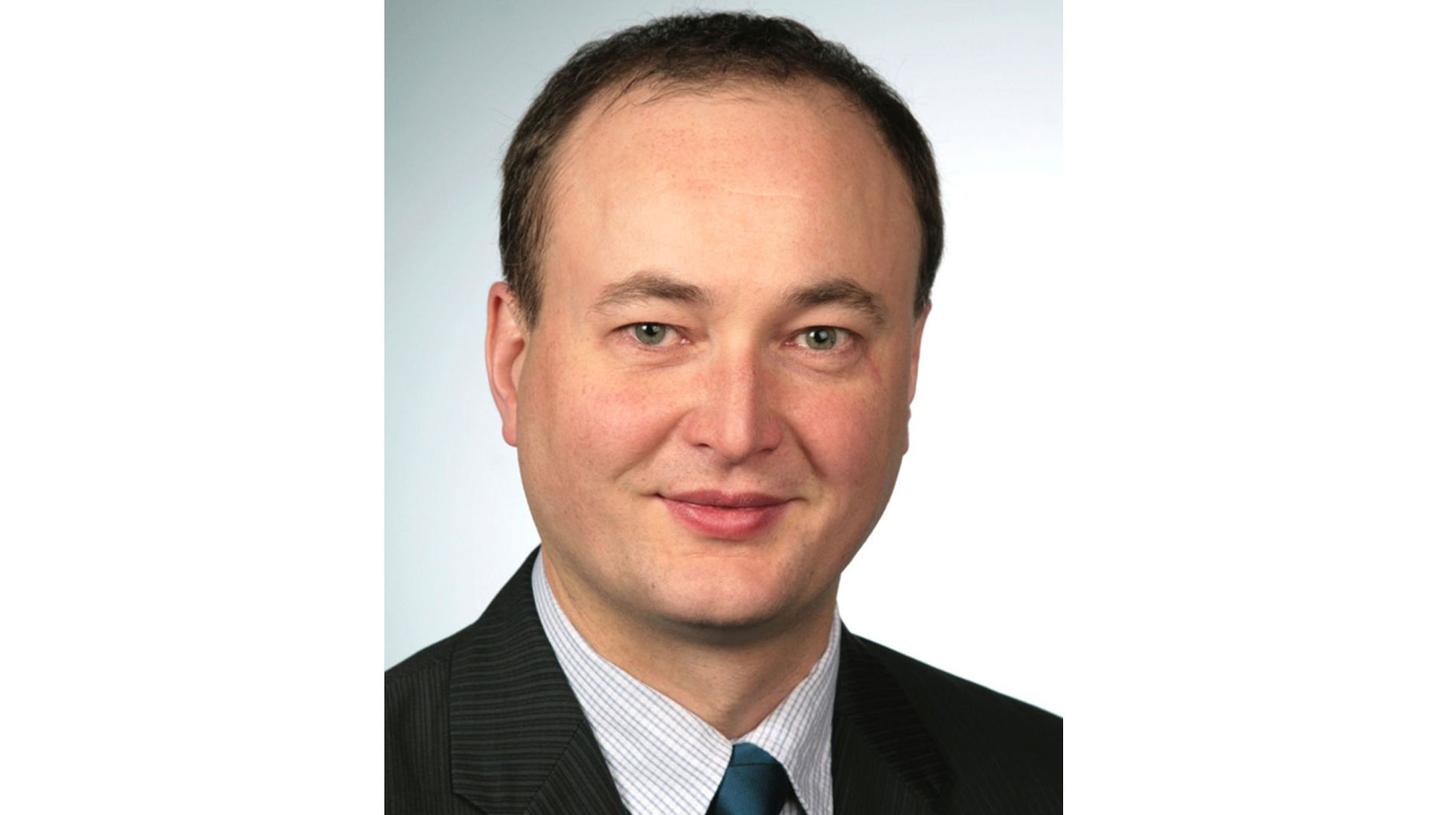
Dr. Heinrich von Busch, PhD, is Product Owner Artificial Intelligence for Oncology at Siemens Healthineers in Erlangen, Germany