- Home
- Solutions informatiques et logicielles
- Artificial Intelligence
- AI campaign
- Changing the way we think about radiation delivery
Changing the way we think about radiation delivery
Adaptive therapy involves the ability to alter a radiotherapy treatment plan based on tumor and anatomical changes over a course of therapy with the goal to better target the tumor, reduce dose to healthy tissue, and potentially improve overall outcomes.
Achieving this has typically required time-consuming re planning between treatment sessions or monopolizing a linac for an extended period while a patient waits on the treatment couch for new plans to be generated.
Ethos® therapy, a radiotherapy treatment system that uses artificial intelligence (AI) and machine learning to accomplish adaptive radiotherapy that is practical and affordable in scale that makes it a viable option for many patients in busy radiation oncology departments.
Available in Ethos® therapy. This product is not available for sale in every market, please contact your local sales representative.
Adaptive radiotherapy
Challenges in the delivery of adaptive radiotherapy
- Performing a full treatment planning workflow during a radiotherapy treatment session while the patient is on the treatment couch is a complicated task and requires time, significant attention and knowledge.
- Manual delineation of target structures during daily replanning is time consuming and technically challenging.
- Producing quality plans using inverse planning, by dedicated treatment planning staff is challenging and the skill and expertise of the treatment planner can have a major impact on the final plan quality.
Removing the barriers to adaptive therapy
The challenges involved in delivering on-couch adaptive therapy are addressed, in the Ethos therapy system, through a re-planning workflow that has been reduced to well-defined and predictable clinical decision points in order to lower the cognitive load of the clinician.
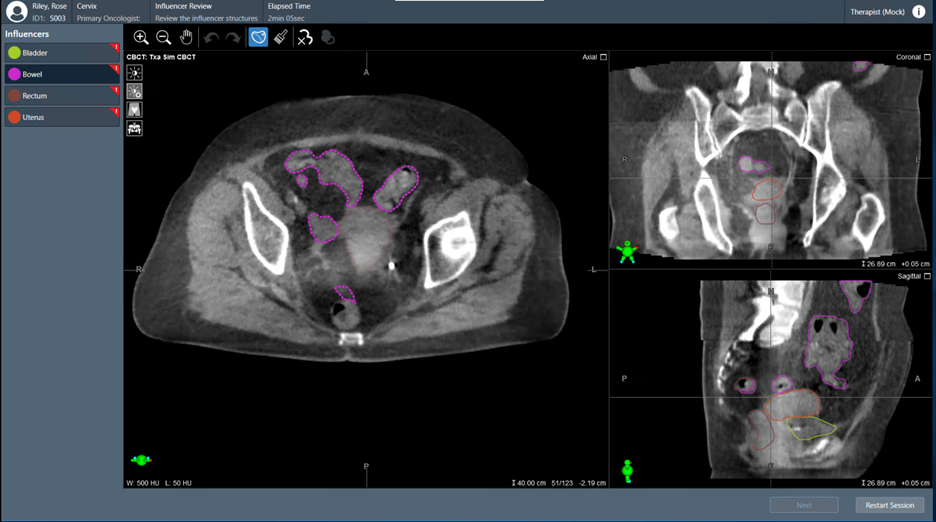
Detection of daily anatomy
To address the challenges involved during detection of daily anatomy, an AI-based algorithm that is based on convolutional neural networks is used to contour normal organ structures which influence the daily shape of the target on the newly acquired kV-CBCT. These “influencer” structures are those structures that are in the closest proximity to the target and have the biggest impact on the shape of common clinical target structures.
On-couch Adaptive Treatment Plan Generation: Intelligent Optimization Engine
Within Ethos therapy, the treatment planning is highly automated to allow the user to focus on the clinical aspects of the patient’s therapy. In order to automate the plan generation, we introduce an algorithm which orchestrates the plan optimization, the Intelligent Optimization Engine. The Intelligent Optimization Engine aims to perform all the actions necessary to generate high-quality dose distributions which meet the clinical expectations for the plan, and ensure the plan is dosimetrically accurate.
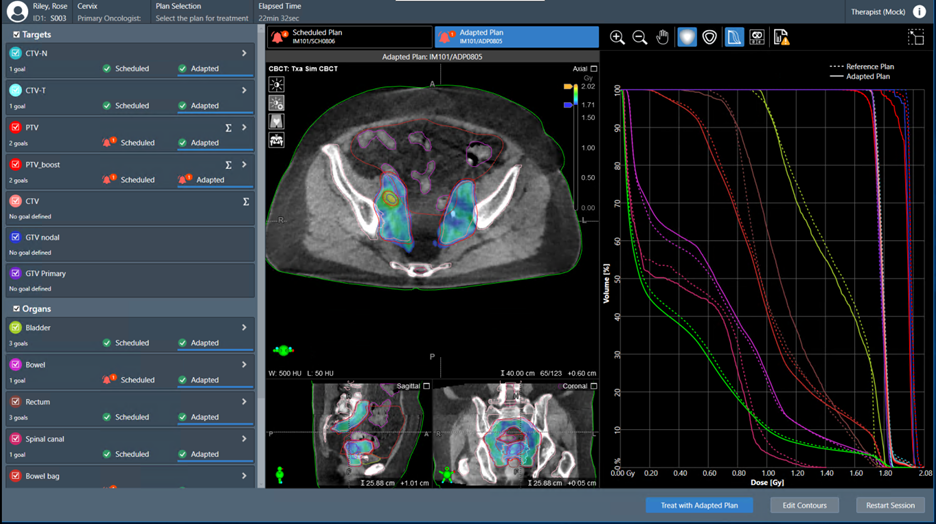
RapidPlan Knowledge-Based Planning
RapidPlan® knowledge-based planning, a machine learning tool, can potentially introduce greater quality and efficiency into treatment planning through the power of historical patient data. The machine learning models within RapidPlan take inputs from dosimetric and geometric parameters of all plans included in its training set. As an output, the models can generate predictions of the dose volume histograms for modeled structures and generate the optimization objectives needed to drive DVHs to those predictions. This permits the clinician to evaluate the predicted normal organ sparing prior to generation of a deliverable treatment plan, as well as incorporate the prediction to the plan quality evaluation.
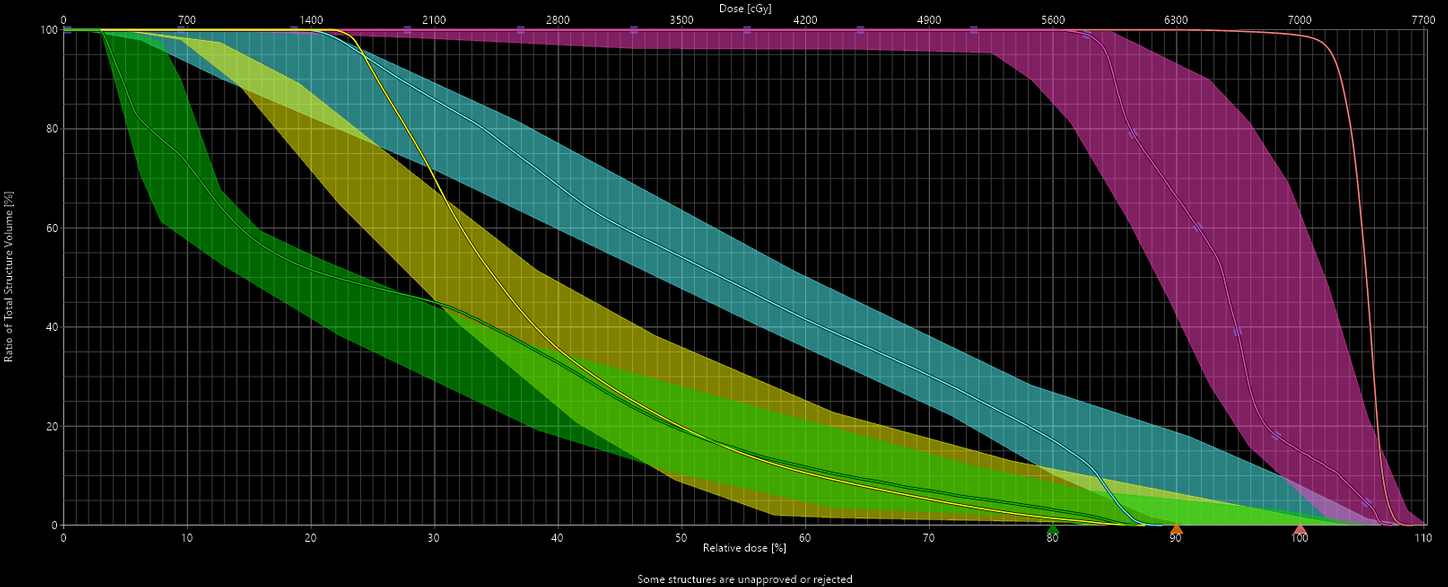
A paradigm shift to scalable adaptive therapy
The Ethos therapy system incorporates technology that uses artificial intelligence and machine learning to create contours and generate adapted plans for clinician review while a patient is on the treatment couch. With guided decision support and fast delivery system, Ethos enables clinics to rethink traditional roles and workflows. Adaptive therapy becomes a practical and accessible treatment option for more patients and brings us another step closer to a world without fear of cancer.
Clinical examples
Prostate and Nodes
02Bladder
02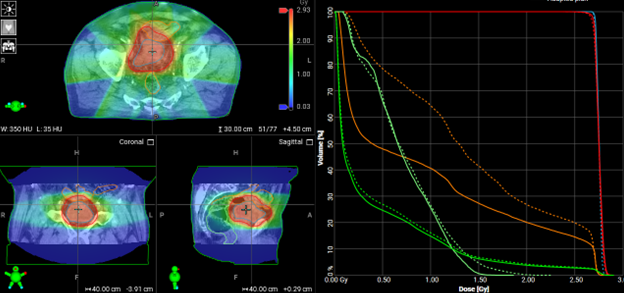
Adapted Plan
Adapted plan was chosen, due to
- Bladder smaller than reference
- Reduced bowel doses with adapted plan
- Adapted plan selected in all adaptive fractions for this patient
Data provided by customer in the UK. These points above are the opinions of this customer, they were not compensated for these opinions and do not necessarily represent those of Varian.
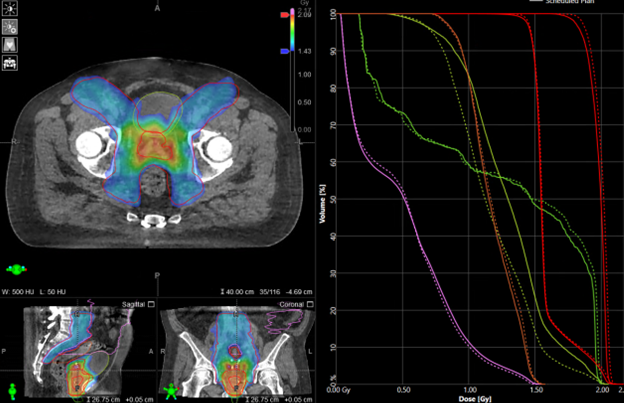
Scheduled Plan
- 9 field IMRT
- 2Gy x 39 fractions to CTVp
- 1.5Gy x 39 fractions to CTVn
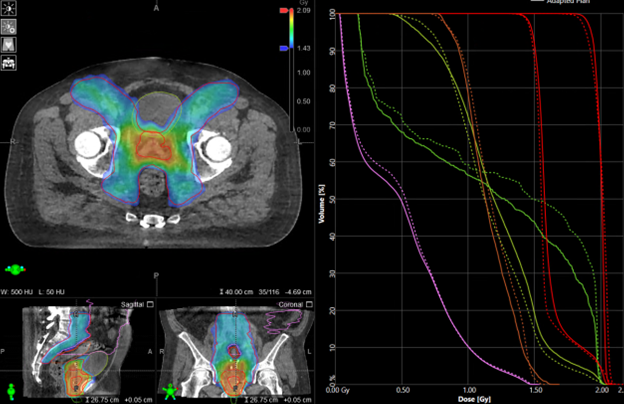
Adaptive Plan
Adaptive plan was chosen due to:
- Better dose distribution
- More clinical goals achieved
- 97% of fractions are superior for the adapted plan compared to scheduled plan
Data provided by Icon Group, Australia. These points above are the opinions of Icon, they were not compensated for these opinions and do not necessarily represent those of Varian.
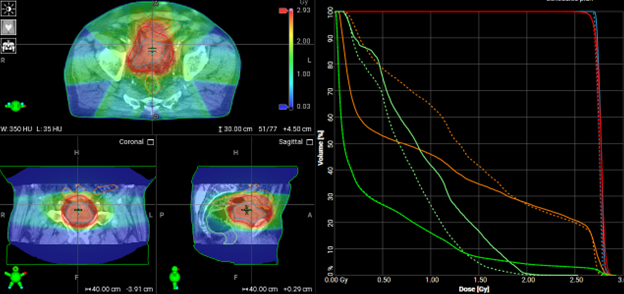
Scheduled Plan
- 7 field IMRT
- 2.75Gy x 20 fractions to PTV
- ~19.5 mins average adaptive fraction time
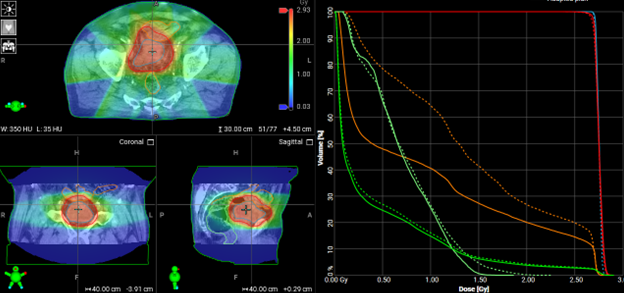
Adapted Plan
Adapted plan was chosen, due to
- Bladder smaller than reference
- Reduced bowel doses with adapted plan
- Adapted plan selected in all adaptive fractions for this patient
Data provided by customer in the UK. These points above are the opinions of this customer, they were not compensated for these opinions and do not necessarily represent those of Varian.
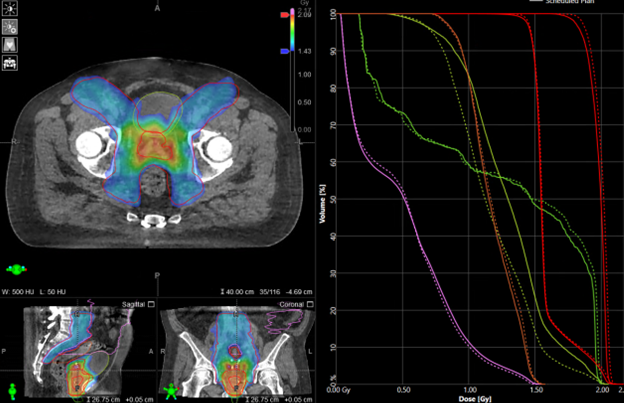
Scheduled Plan
- 9 field IMRT
- 2Gy x 39 fractions to CTVp
- 1.5Gy x 39 fractions to CTVn
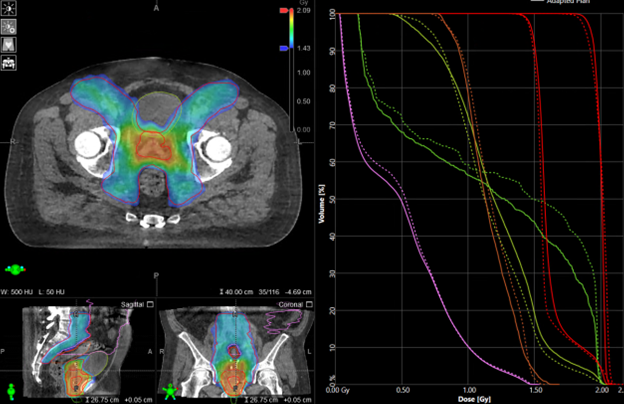
Adaptive Plan
Adaptive plan was chosen due to:
- Better dose distribution
- More clinical goals achieved
- 97% of fractions are superior for the adapted plan compared to scheduled plan
Data provided by Icon Group, Australia. These points above are the opinions of Icon, they were not compensated for these opinions and do not necessarily represent those of Varian.
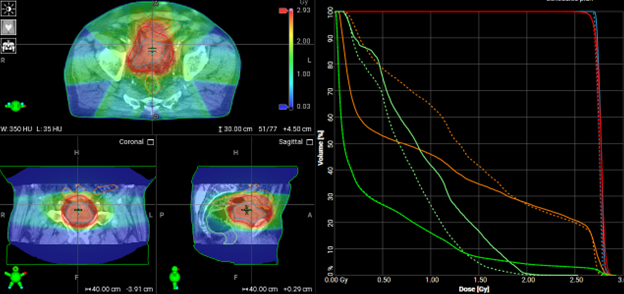
Scheduled Plan
- 7 field IMRT
- 2.75Gy x 20 fractions to PTV
- ~19.5 mins average adaptive fraction time
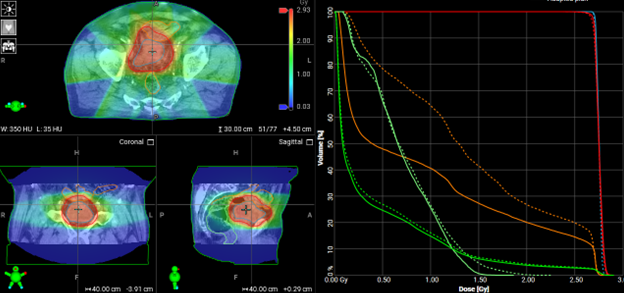
Adapted Plan
Adapted plan was chosen, due to
- Bladder smaller than reference
- Reduced bowel doses with adapted plan
- Adapted plan selected in all adaptive fractions for this patient
Data provided by customer in the UK. These points above are the opinions of this customer, they were not compensated for these opinions and do not necessarily represent those of Varian.
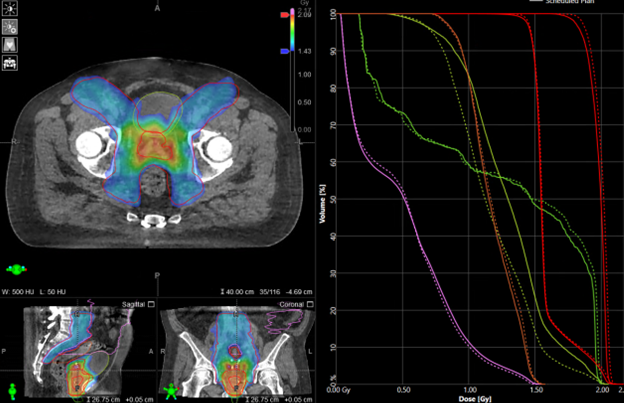
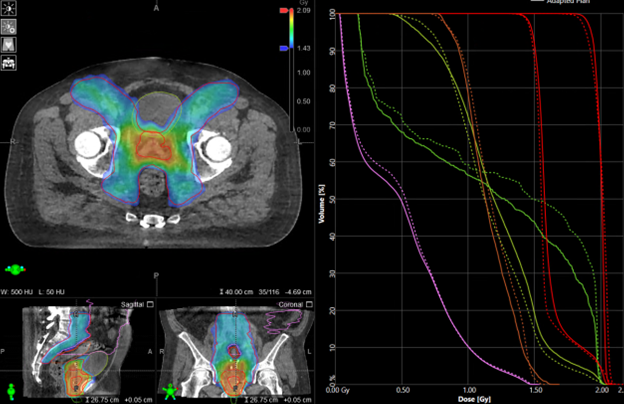
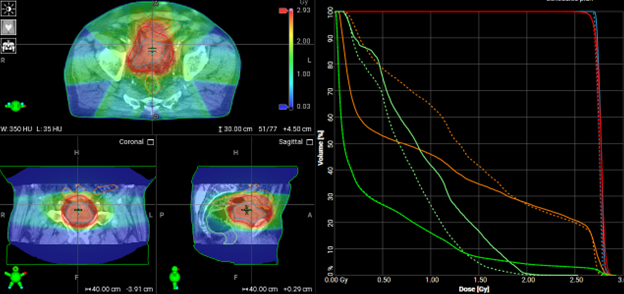
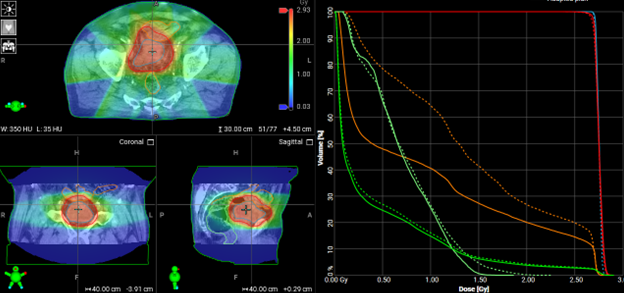
Customer experiences
“In a field where time is key, Ethos’ online adaptive treatment makes use of artificial intelligence to fasten organs delineation process on daily CBCT images. Decreasing the time needed for adaptive radiotherapy not only minimizes anatomy variations but also opens the door for its widespread use without the need for patient selection scheme.”
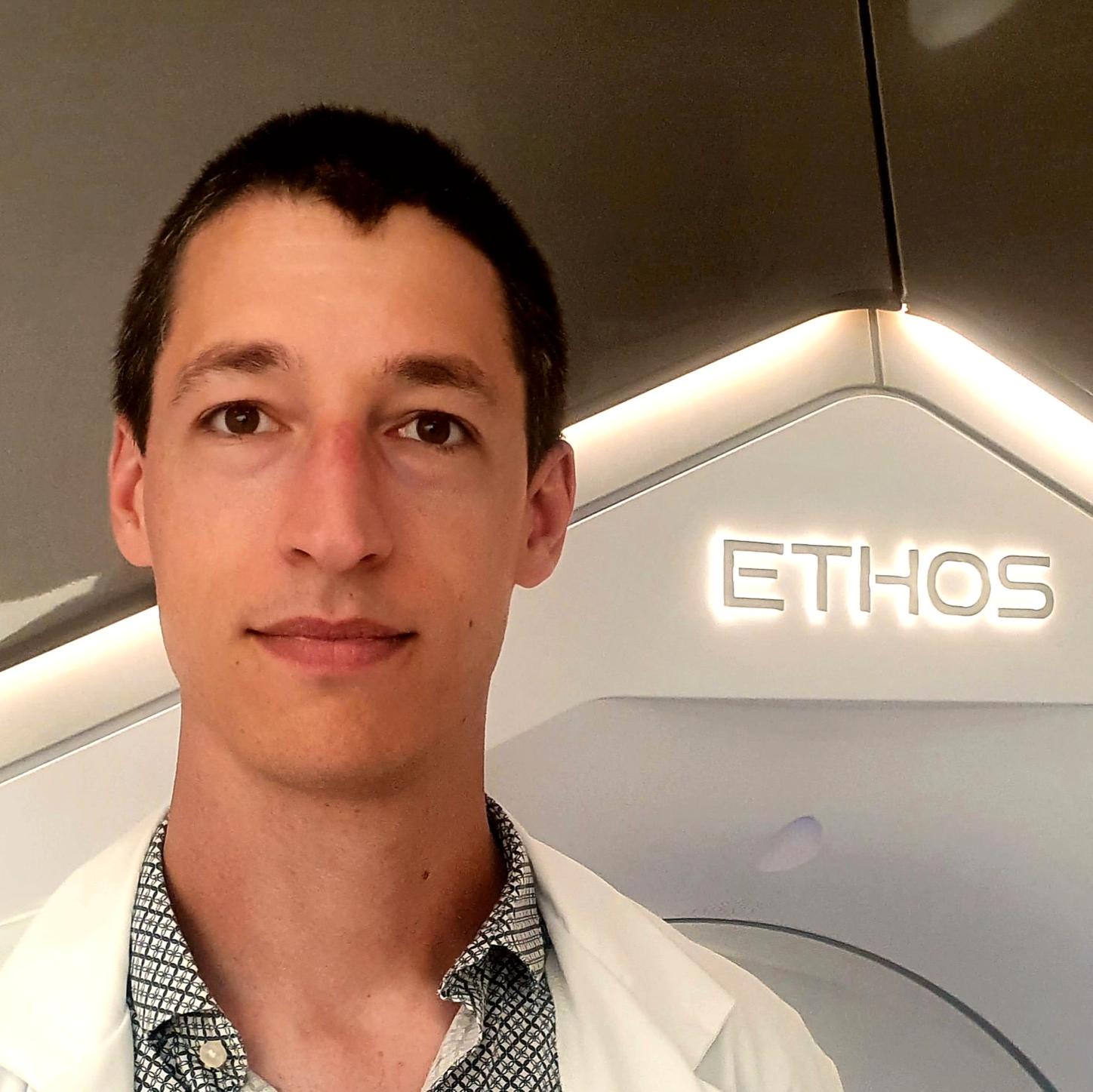
Dr. David Dechambre,
Saint-Luc UCLouvain, Bruxelles
Contact
See the other topics here