Home
Perspectives
- From Automating Muscle to Augmenting the Brain
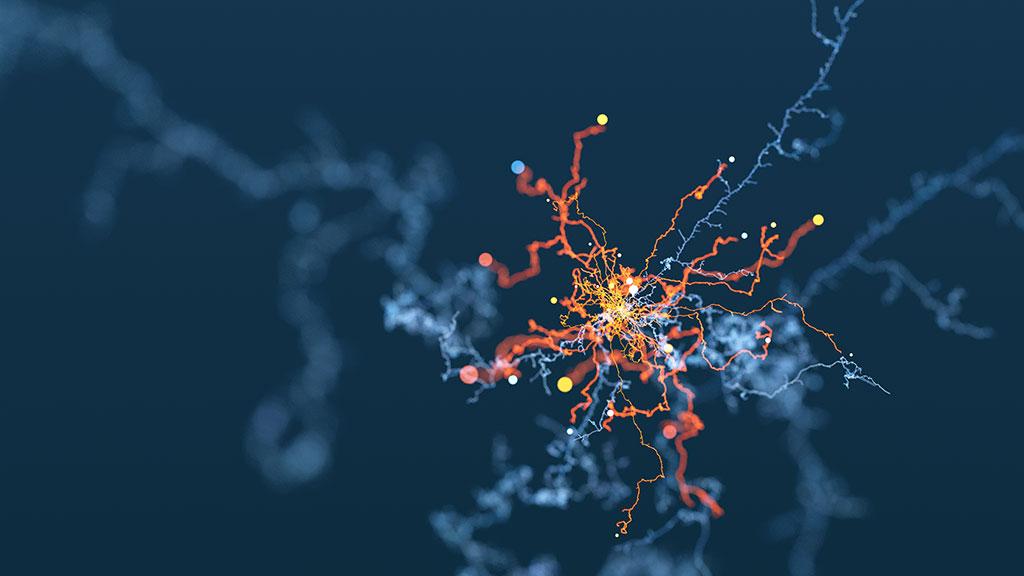
From Automating Muscle to Augmenting the Brain
Download this white paper as PDF
An exciting new survey from Siemens Healthineers of 200 hospital or laboratory senior executives and lab directors explored the not-so-distant future of the impact of artificial intelligence (AI) on the in vitro diagnostic (IVD) laboratory.
- 69 percent expect widespread adoption of AI in the IVD lab within the next four years.
- 92 percent expect AI to have a significant impact on healthcare eventually.
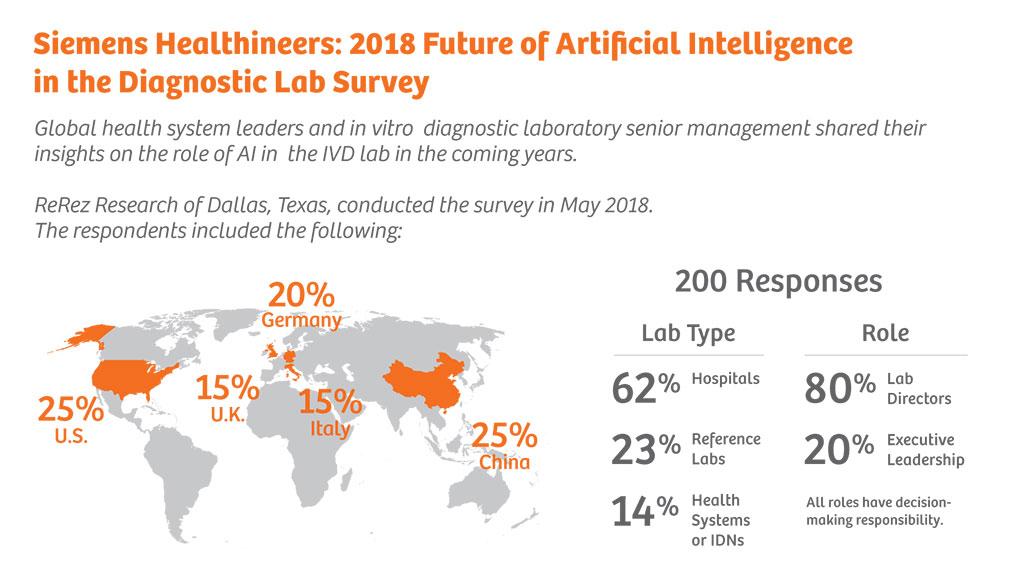
2018 Future of Artificial Intelligence in the Diagnostic Lab Survey
This is good news for patients and providers, as AI is expected to:
- Reduce cost
- Improve the patient experience
- Increase efficiency industrywide
To better envision these AI trends and expectations, let’s understand what AI truly is and how the industry got here.
AI has the ability to transform care delivery: From improved diagnostic accuracy to better patient care, it will propel the industry forward. But AI is a daunting topic—the vast changes, unknown risks, and uncharted territory can overwhelm experts and novices alike. Still, this type of change isn’t unprecedented in the industry; the introduction of X-ray technology in the late 19th century evoked many of the same fears yet ultimately transformed the healthcare industry.
X-rays Herald New Era of Medical Technology
In 1895, German physicist Wilhelm Roentgen discovered X-rays and used the new technology to scan his wife’s hand. Seeing the photo of her skeletal fingers, she remarked, “I have seen my own death.” X-rays became an instant medical phenomenon, allowing doctors to see what they’d never seen before. For the first time in history, doctors could evaluate internal signs, leading to a major leap forward in diagnostic capability.
But this initial fascination began to give way to two fundamental fears: invasion of privacy and unintended consequences. But, the clear value that X-ray imaging provided to healthcare pushed the industry to address privacy and safety challenges, and X-rays continue to play a vital role in medical imaging today.
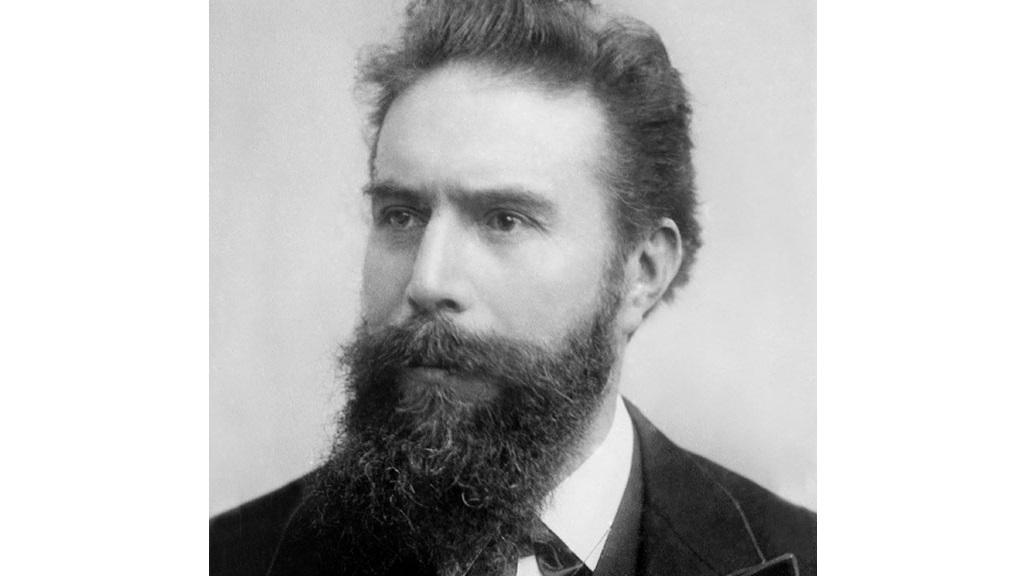
Healthcare is on the precipice of a major leap forward: artificial, or augmented, intelligence.
Many of the same trepidations persist, from concerns for patient confidentiality and privacy to fear of unintended consequences that come with machines that will become more intelligent than humans. Yet, as with X-rays, AI will transform healthcare in fundamental ways.
In this white paper, we will:
- Discuss what AI is and why it is relevant for IVD laboratories.
- Examine the road to AI.
- Consider how regulation and reimbursement will impact its adoption.
- Provide tangible actions that organizations can take today to prepare for this emerging technology.
What Is AI?
For the purposes of this white paper, focusing on healthcare and specifically the IVD lab, we define artificial intelligence as follows:
AI refers to sophisticated software systems that enable computers to augment, or even emulate, human intelligence and decision making.
Beneath the heading of AI is “machine learning,” which uses algorithms to parse and learn from data and then applies this learning to provide insight and make informed recommendations. The system learns by mimicking the perception and decision making of experts.
By analyzing large amounts of training data that has been curated with known outcomes (such as oncological imaging where the diagnosis is known), the system recognizes patterns and applies what it has learned to new scans, automatically perceiving and analyzing signs to make determinations.
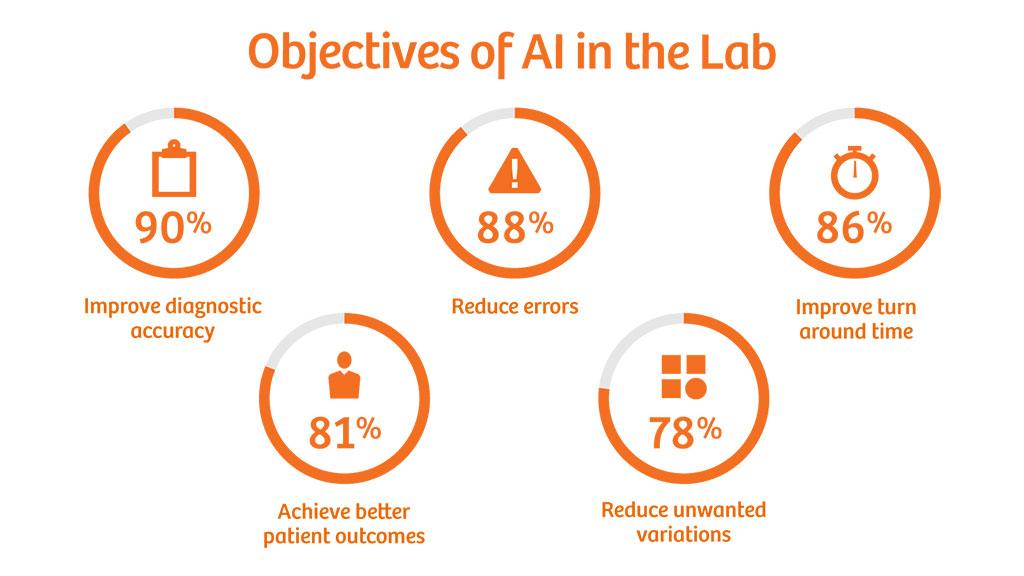
If the algorithm makes an error in detection or diagnosis, it can be corrected by the expert. Subsequent versions of the algorithm can then learn from these mistakes.
The key distinction between AI and other software or computer-based technologies is that AI has the capacity to learn and improve from data and experience. Other technologies can handle complex tasks but cannot perform actions or form conclusions to augment clinicians’ and patients’ care decisions that are not specifically programmed.
In healthcare, the massive datasets used in training, validation, and subsequent continuous learning may originate from:
- Clinical records
- Population electronic health records
- Medical research
- Laboratory information systems (LIS) data
- Diagnostics such as scans, imaging, and video
Most Believe in AI’s Impact, But Are Unsure Where to Begin
In the 2018 Artificial Intelligence in the Diagnostic Lab survey:
- 69 percent believe that AI will be implemented in the clinical lab within the next four years.
- 54 percent do not know where to begin.
In fact, the path to AI has already begun.
- 49 percent of all labs are already engaged with AI.
- 34 percent are planning to engage with AI in the future.
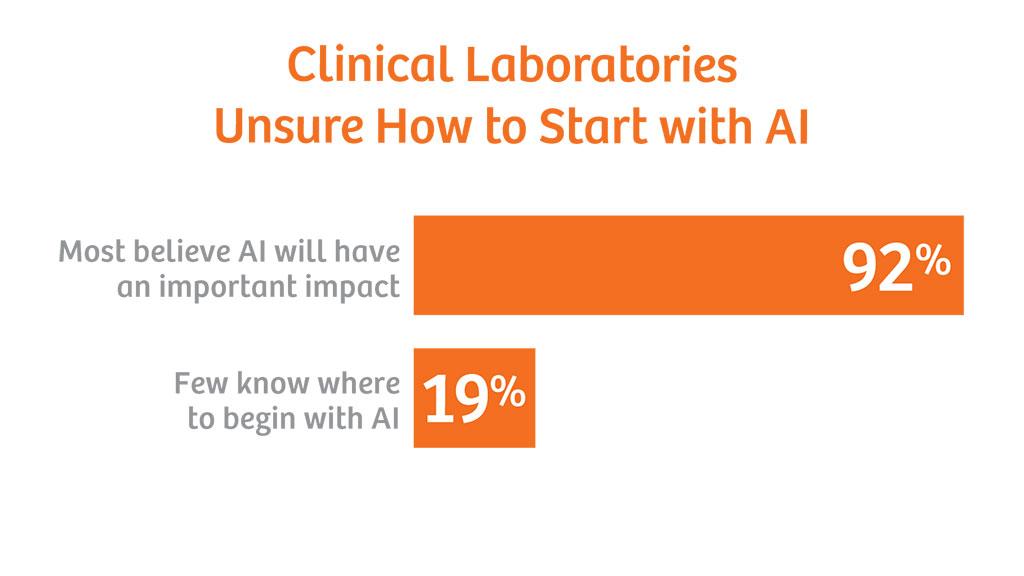
“I definitely think AI will make an impact, but I think it’s early. We’re utilizing algorithms to identify patients who have care gaps in their treatment plans. It’s not quite AI—it is more an early precursor. In the future, we’re going to see a lot more sophistication with AI in identifying what patients need.”
Eric Carbonneau, Director Core Laboratory Operations, TriCore Reference Laboratories, United States
But, as Carbonneau states, we are still in the early days.:
- Only 20 percent are at the implementation stage.
- Only 29 percent are discussing or trialing AI.
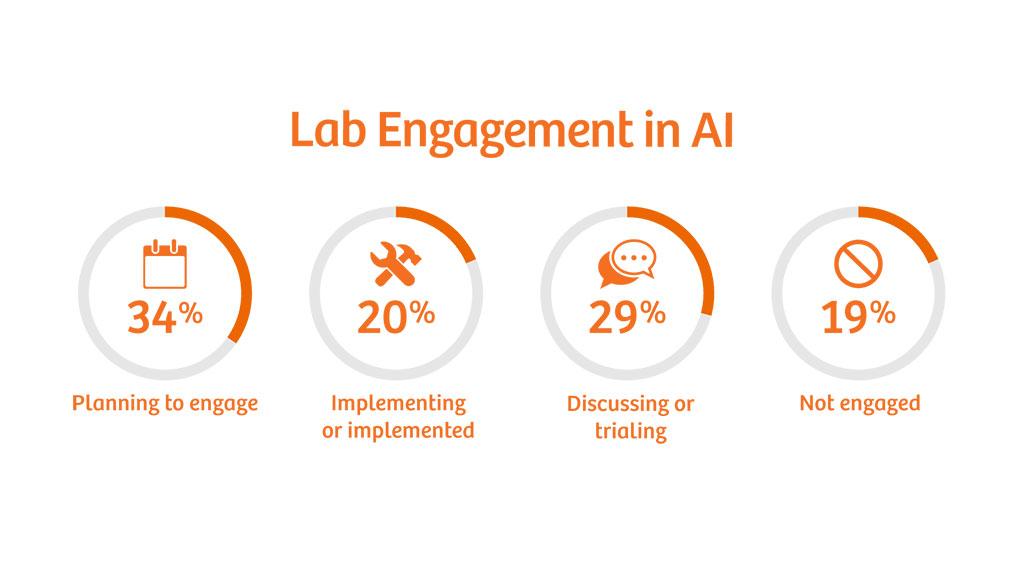
Survey Results: Lab Engagement in AI
Automating Laboratory Diagnostics: the Road to AI
Stage 1: Automation replaces manual labor
Investment in innovative laboratory technology such as automation has reduced manual labor and increased efficiency throughout the diagnostic testing process. Most, if not all, hospital and reference laboratories have already implemented some form of automation.
Stage 2: Digitalization automates information flow
Rules-based programming has replaced many human tasks and judgment decisions in the clinical laboratory, accelerating workflow and reducing errors. Digitalization has replaced handwritten results manually compiled and forwarded to physicians. Technology has evolved to include:
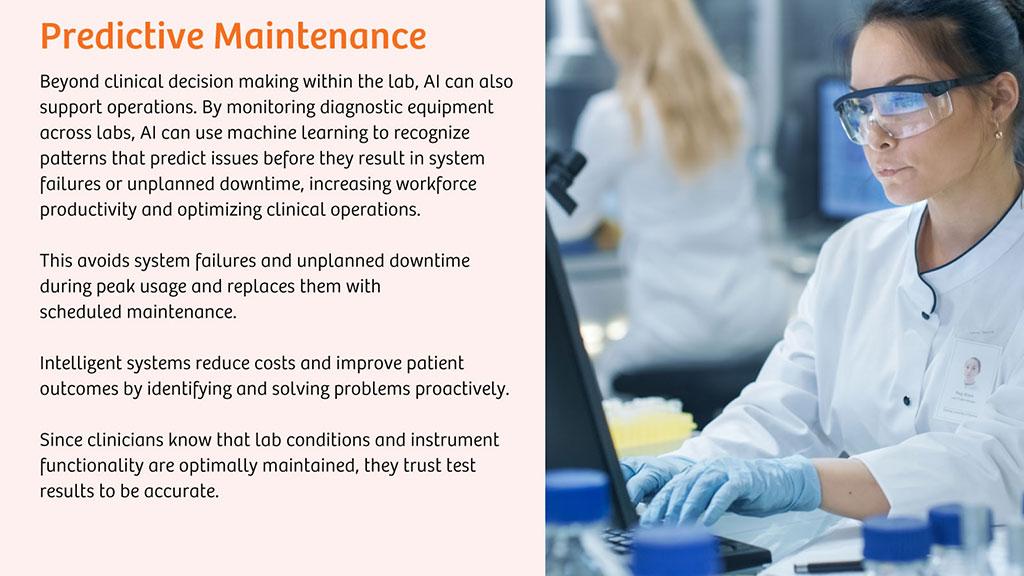
Predictive Maintenance Can Anticipate Issues Before They Result in System Failures
- Automated test ordering
- Autoverification
- Workflow management
- Intelligent tube routing
- Clinical decision support
Autoverification enables consistent and accurate confirmation of test results without human intervention. It increases efficiency and reduces human error by having software perform a time-consuming, repetitive task, significantly increasing workforce productivity
Additionally, predictive maintenance technology reduces critical downtime for key analyzers by constantly monitoring systems and proactively determining the optimal time for repair before an unexpected problem can disrupt operations.
Diagnostic Decision Support System
Computer-aided detection and diagnosis uses algorithms to analyze the data available in a patient electronic health record (EHR), lab test results, diagnostic imaging, etc., to suggest possible diagnoses to the clinician, which improves accuracy by almost 9 percent.[1]
This technology is known as a diagnostic decision support system (DDSS). One example is automated cardiac risk assessment, which uses global cardiac risk scores in combination with lab data and EHRs to computerize risk stratification. As both the quality and quantity of available data increase through careful curation, the reliability of automated diagnostic and therapeutic recommendations will continually improve.
A DDSS lowers costs and improves patient outcomes in three primary ways:
- Recommends the ideal mix of tests to determine a diagnosis.
- Automates mundane aspects of the clinician’s job, allowing him or her to prioritize more-critical tasks.
- Delivers faster and more accurate diagnoses and treatments with improved algorithms.
The purpose of the DDSS is not to replace diagnosticians, but rather to augment their decision making.
“There’s a lot to expect in the coming 2–3 years. AI will be used for laborious and dull types of work that can be easily automated without interfering with the real expertise of the doctors. There will still be a lot of work done by doctors, but it will be a different type of work. It will focus on their real expertise, which can’t be easily translated to AI programs.”
Warry van Gelder, Medical Director, Result Laboratorium, Netherlands
Stage 3: Lab leaders see AI in the near future
Healthcare—and the diagnostic lab in particular—is rapidly approaching AI. Of hospital executives and lab directors surveyed:
- 69 percent anticipate implementation in the lab within four years.
- 88 percent predict AI will be important for the diagnostic lab.
Survey respondents envision better patient-care pathways, improved detection and diagnosis of rare conditions, and better prevention of chronic diseases.
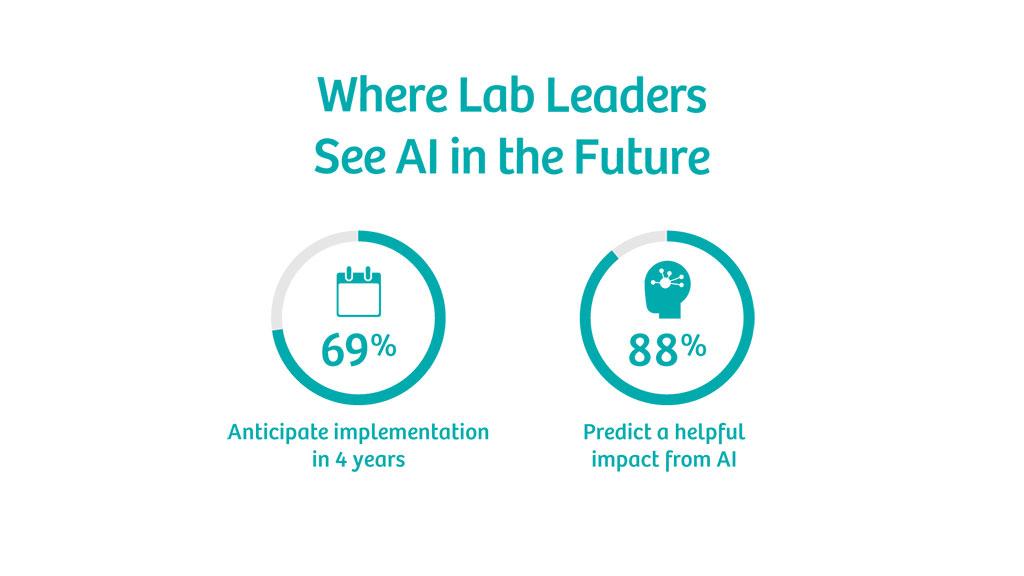
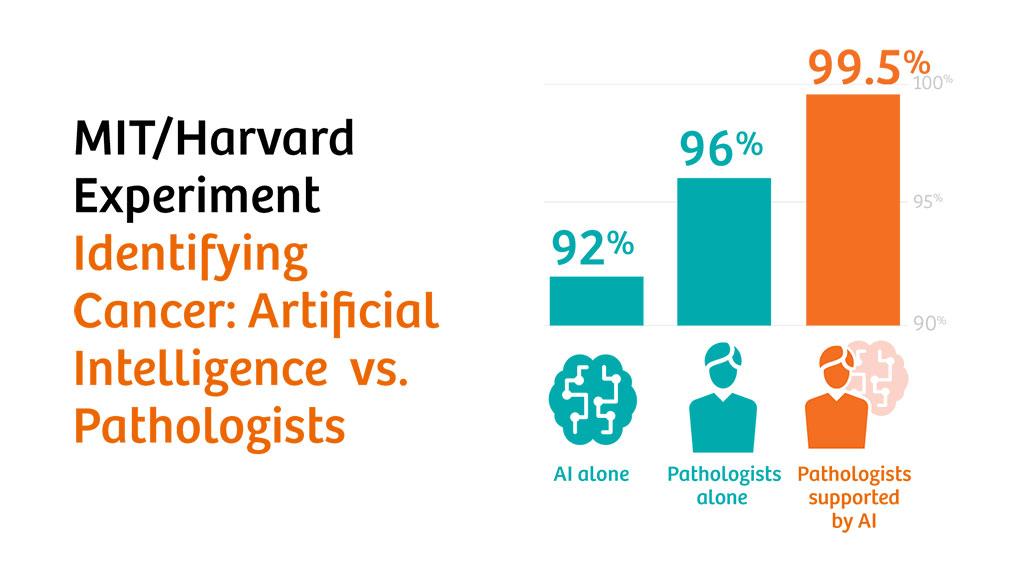
Pathologists Supported by Artificial Intelligence Have Higher Diagnosis Accuracy
Early AI processes will reduce or eliminate manual effort and speed decision-making work but are not expected to fully replace the physician and lab technologist. Instead, AI will support laboratory clinicians by streamlining the mundane aspects of their day, enabling them to focus on the complex cases.
To illustrate the potential, consider the results of a joint MIT/Harvard experiment from 2016.[2] The team evaluated the performance of three groups in correctly identifying cancerous lymph nodes:
- Pathologists alone
- AI alone
- Pathologists supported by AI
Individual pathologists outperformed AI, correctly identifying 96 percent versus 92 percent of diseased nodes. However, when the pathologists were supported by AI through computer-aided detection/diagnosis, they were able to accurately diagnose 99.5 percent of the cases.
The team determined that most of the errors made by the pathologists were due to time constraints, and when AI quickly eliminated simple cases, the humans could take their time on complex scans and ensure accuracy.
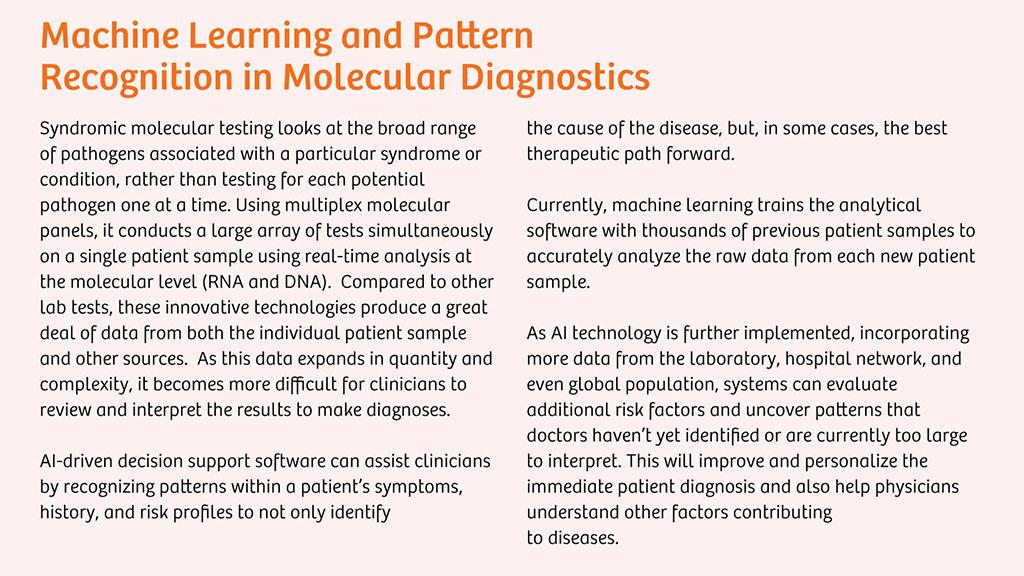
Machine Learning and Pattern Recognition in Molecular Diagnostics
Stage 4: AI becomes mainstream
Eventually, as it continues to learn and improve, AI will handle more non-patient interactions. AI is expected to transform the full patient-care continuum, from checking a patient’s EHR to ordering tests and even integrating diagnostic information, symptoms, risk profiles, and demographics to recommend diagnoses and treatment options.
Survey respondents offered insight into their expectations for AI:
- 90 percent: Valuable impact on the diagnostic process within the next decade
- 88 percent: Reduction in errors
- 86 percent: Improved TAT
- 81 percent: Better patient outcomes
- 78 percent: Fewer unwarranted variations
Cost, lack of training, and regulation are barriers to mainstream adoption of AI. Implementation will require both patients and the medical community to develop confidence in AI’s findings as well as effective oversight and approvals from regulatory agencies.
Let’s examine the current state of regulation and project how it may change in the next few years.
In cases of discrepancy between test and diagnosis results, AI may automatically find these exceptions and remove errors and redundant information by analyzing large amounts of data.
Dr. Ling Qiu, Vice Director, Department of Lab Medicine, Peking Union Medical College Hospital (PUMCH), China
The Evolution of Regulation
Healthcare leaders have expressed concern about the impact of regulation on AI’s adoption: 81 percent believe that regulation will be a significant hurdle. Much of this concern stems from the current state of regulation, which is evaluating AI in the same way as traditional technologies.
The existing regulatory-approval framework was developed for “static” software and requires that each new release be submitted for review. But AI systems are inherently dynamic and continuously evolving, with their ability to autonomously analyze and learn from information and use this data to make correlations, predictions, and decisions.
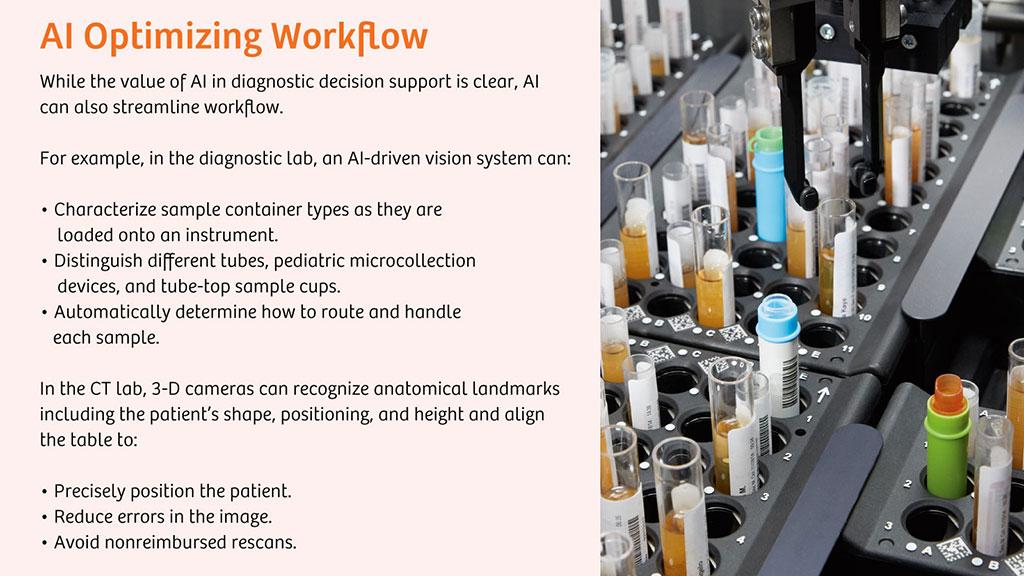
Data privacy laws affect how systems access and share data, which impacts AI’s ability to learn. For example:
- Health Insurance Portability and Accountability Act (HIPAA) in the U.S.
- General Data Protection Regulation (GDPR) in Europe
Recognizing the potential for AI advancement, global regulators are exploring how to balance patient safety and confidentiality with the need for innovation. For example, the U.K.’s Committee on Artificial Intelligence has tasked the National Health Service (NHS) to outline data sharing plans by the end of 2018.[3]
In the U.S., the Food and Drug Administration (FDA) has for the past 20 years approved computer-assisted detection and diagnostic devices possessing progressively increasing degrees of autonomy.
Recently, the FDA approved AI-driven healthcare tools to interpret:
- Computed tomography angiography (CTA) images for indications of a stroke
- Ophthalmic scans for diabetic retinopathy
- X-rays for hard-to-identify wrist fractures
The FDA has also introduced the Digital Health Innovation Action Plan to foster innovation while continuing to protect public safety.
Given the current regulations, it is important to focus on incremental improvements. As Dr. Ankur Kapoor, director of Vision Technologies and Solutions at Siemens Healthineers, states, “In these early days, AI is better with things like automation, where you don’t need to be perfect, just better.” As regulations evolve and pioneering programs become accepted across the industry, organizations that have introduced early-stage AI will be better prepared to holistically adopt the technology.
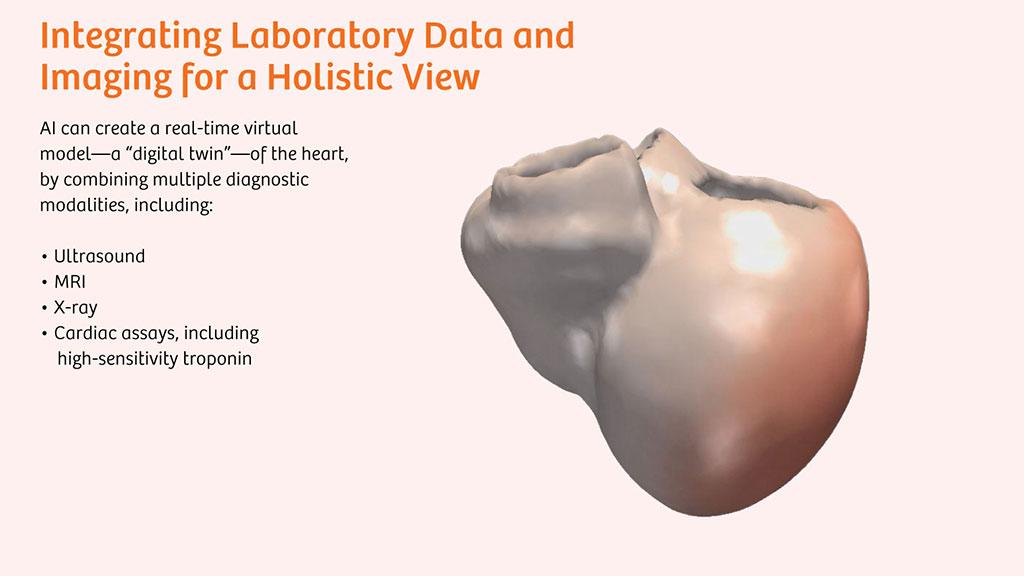
Who is to blame when AI makes a mistake? We can’t start early enough in discussing these issues.
Warry van Gelder, Medical Director, Result Laboratorium, Netherlands
Ultimately, regulation will catch up with the new technology—it always does. X-rays were overregulated in 1913,[4] but scientists and regulators worked together to introduce progressive radiation-dose reduction and ease restrictions, creating standards that are still in use today. As with X-rays, the promise of AI’s impact on the field of healthcare will drive cooperation between regulators and AI developers to implement the technology safely without restricting innovation.
In addition to regulation, reimbursement is critical to the adoption of AI. The reduced costs, increased efficiency, and improved outcomes enabled by AI can be realized only if providers implement innovative products and services. Both public and private payers need to incentivize adoption of AI through changes in reimbursement policies.
How to Prepare for AI
To effectively prepare for AI, changes must occur at both the industry and organization level. AI needs large sets of data from which to learn and adapt, and no individual organization will be able to supply a sufficient volume of information. As one example of the scale that is necessary, in the U.S., the National Institutes of Health (NIH) All of Us Research Program aims to gather data on a diverse million-person cohort to customize care and improve diagnostic accuracy by expanding precision medicine.
For AI to thrive, the healthcare industry must come together as a whole to move toward data sources that are:
- Accessible
- Interoperable
- Standardized
- Curated
Best practices should be observed to avoid potential bias within the data that can skew diagnostic conclusions. It is also vital to ensure that data is anonymized, securing the privacy of protected health information without losing the usefulness and integrity of the data.
In parallel, individual organizations should prepare for their internal implementation of AI and educate key decision makers on its value.
Cybersecurity should not only be a top priority to protect patient data, operations, and IT systems, but also an integral part of system design.
Industry changes
The entire healthcare industry will benefit from changing the way data is formatted and collected.
Sixty-six percent of executives and directors believe that lack of standardization will pose significant challenges in implementing AI.
Currently, data is siloed within individual departments, hospitals, or healthcare systems, often in proprietary or irregular formats. This restricts AI’s training, validation, adoption, and continuous learning process.
By standardizing the data structure and access that link healthcare systems and population data both nationally and globally, the industry will enable individual systems and networks to access and implement transformative AI applications.
By participating in steering and standards-setting groups, healthcare leaders can help guide the discussion and use this interaction to define their own internal strategies and tactics. Such groups include:
- AdvaMedDx
- Precision Medicine World Conference (PMWC)
- Medical Imaging & Technology Alliance (MITA)
- Global Medical Technology Alliance (GMTA)
Organizational changes
To best prepare for emerging AI, healthcare organizations should educate key decision makers as well as modernize their current technology. To avoid a technical skill gap when implementing AI, organizations should establish a plan to train existing staff and enact a hiring strategy to bring on new people with the right skills. By the time the technology becomes more widespread, organizations will be primed for a smooth integration and upgrade process.
To further mitigate the challenges inherent with migrating to any new technology, organizations should plan a roadmap to modernize their current technical capabilities (automation, digitalization, etc.) to set the stage for AI implementation.
AI will increase the prestige of laboratory diagnostics because we will be providing more accurate data. If big data is used together with laboratory data, it will gain more importance.
Mustafa Serteser, Professor, Acibadem University, Turkey
Picturing an Exciting Future
Artificial intelligence will:
- Reduce costs
- Increase diagnostic accuracy
- Improve the patient experience
- Enable a connected clinical laboratory from beginning to end
Dr. Ankur Kapoor of Siemens Healthineers envisions this AI-driven future: “AI streamlines workflow, improves throughput, and improves efficiency for doctors and technicians so they can focus their expertise where it matters.” Just as automated systems and intelligent software have replaced mundane human effort, AI will soon exceed human intelligence, offering insight that can transform care delivery.
Learn more from our executive white paper series:
- Investing in the clinical lab can improve the patient experience and reduce costs. Read The Diagnostic Lab – The Hidden Jewel in the Health System
- How can your lab keep up with increasing workloads despite shortages of qualified staff? Read Increasing Workforce Productivity in the Diagnostic Laboratory to learn about the technology, techniques, and training to help your organization meet the demand.
Related Links
Share this page
- [1] PSNet. https://psnet.ahrq.gov/resources/resource/30828/diagnostic-accuracy-of-gps-when-using-an-early-intervention-decision-support-system-a-high-fidelity-simulation
- [2] Harvard Medical School. https://hms.harvard.edu/news/better-together
- [3] Digital Health. https://www.digitalhealth.net/2018/04/house-of-lords-ai-nhs/
- [4] National Center for Biotechnology Information. https://www.ncbi.nlm.nih.gov/books/NBK232703/
- The concepts and information presented in this paper are based on research study sponsored by Siemens Healthineers and from Siemens Healthineers personnel expertise in this field. These statements are not guarantees of future performance. Opinions quoted by individuals are their own.