Home
Perspectives
Humanizing medtech
Humanizing medtech: The dawn of a digital twin
Although there have been some initial successes in this area, the concept imposes particular requirements on two mammoth tasks in particular: the collection and exchange of data.
Can an algorithm calculate the physical damage caused by eating a bag of chips and the benefits of drinking a green smoothie instead? Can it do so in the form of a personalized prognosis for each individual person?
These questions are the subject of research by scientists from the Weizmann Institute of Science in Rehovot, Israel.
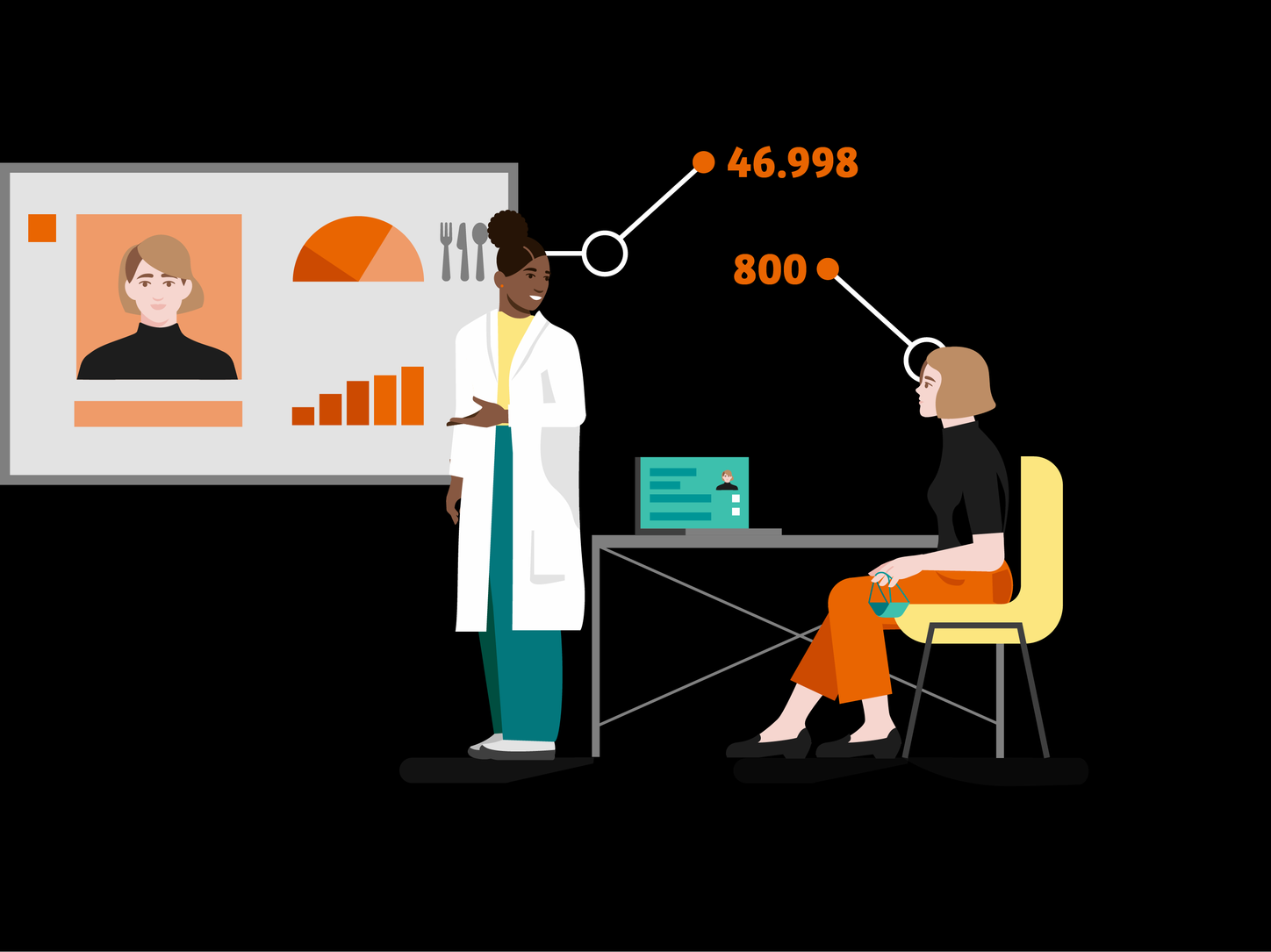
Theoretical scenarios in real time
Reinhard Laubenbacher from the University of Florida proposes using a very similar technique in the fight against viral infections such as COVID-19.2 The researcher is confident that if one were to collect as many pieces of individual patient data as possible and use a computer to run through various models of disease progression, it would be possible to reveal future scenarios in real time.
Systems biologists like Laubenbacher are attempting to gain a fundamental understanding of the relationships between cells and organs. These relationships can be revealed by arranging data systematically in order to deliver key insights that allow the development of innovative medical technology or medicines.
Systems biology
The programming of “digital twins”3 to deliver sensible prognoses is fundamentally reliant on data – lots of diverse pieces of data. Moreover, the data packet must be topped up constantly so that the digital twin can be used for a steady stream of new simulations.
As virtual representations of a real product or process, digital twins always include basic information about the characteristics of their real doppelganger. The concept is already being put to use in industry, where manufacturers can now track every stage of development using a virtual representation. Accordingly, they can test a new or modified product on the heart and kidneys at a very early stage before wasting any material resources.
Digital twin
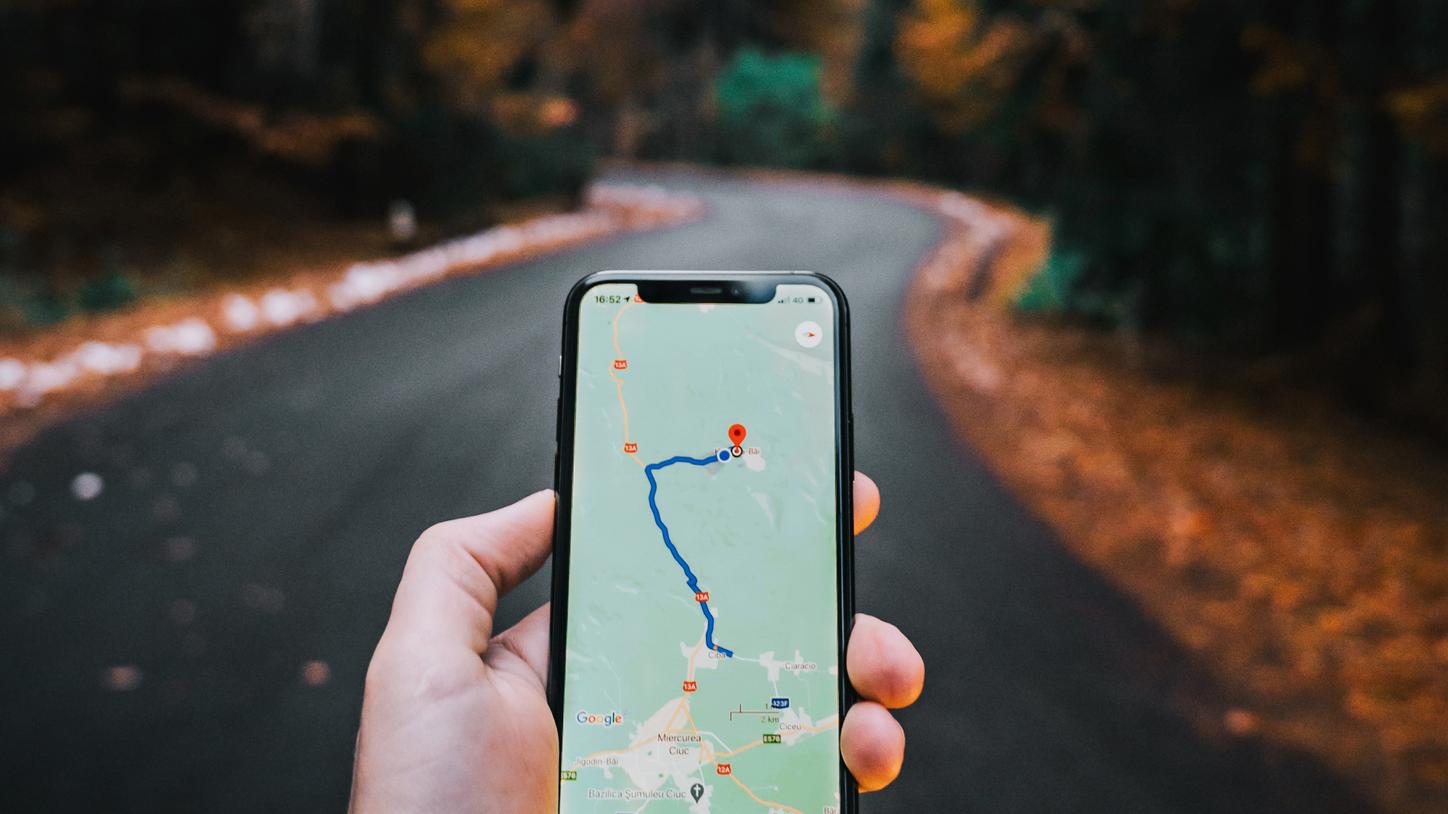
An intelligent guide instead of a map
Most of us consult a digital twin almost every day – and constantly feed it with data – in the form of Google Maps. On this digital map, millions of pieces of real-time information leave a “digital shadow” of current traffic conditions, which is essential in order to provide users with dynamic route suggestions.
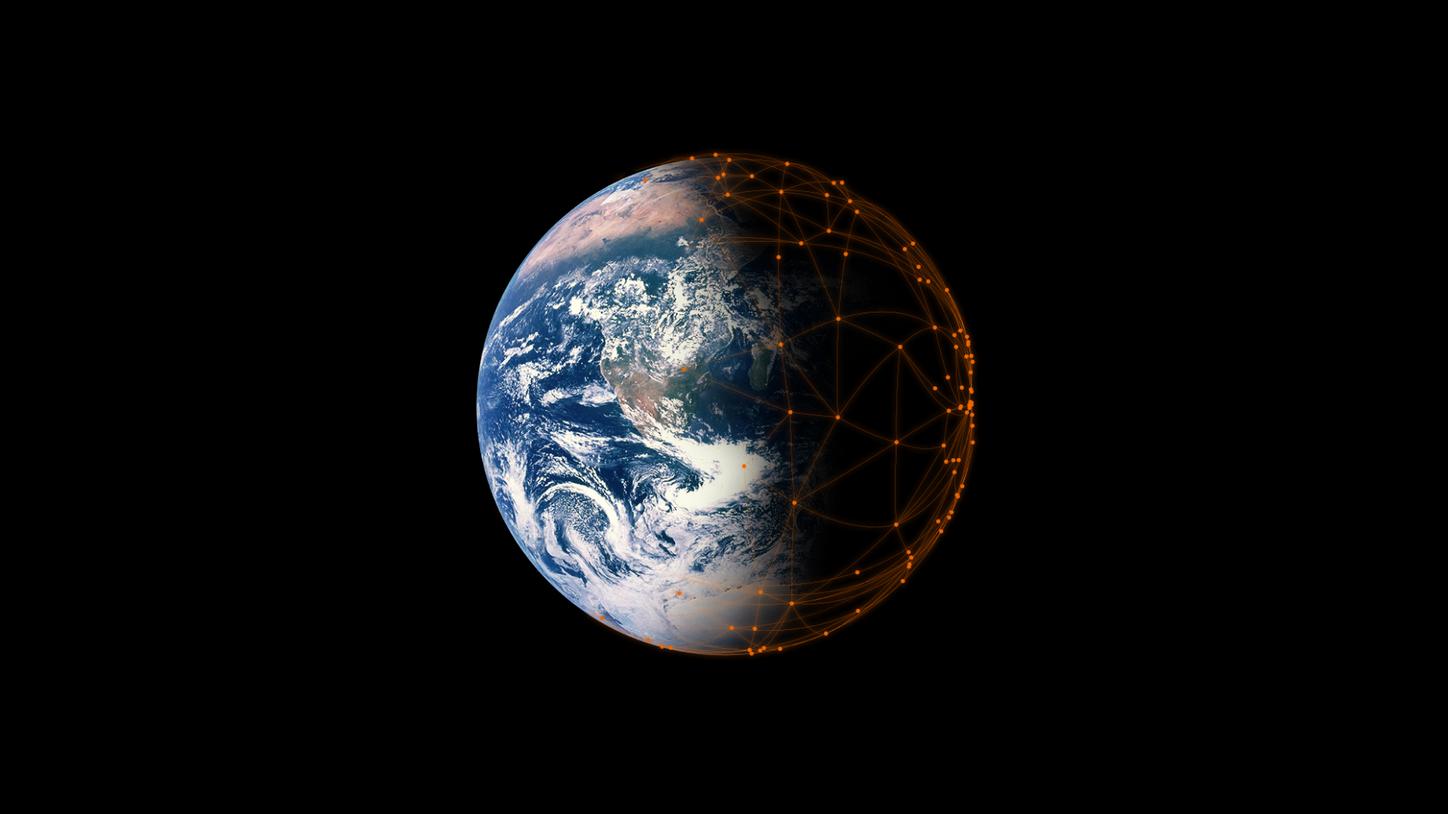
Visualizing the impact of climate change
The Destination Earth initiative aims to create a digital doppelganger of the Earth on supercomputers in order to demonstrate how changes in climate affect our civilization. Insights from this EU project are intended to provide a sound basis for policy-making.
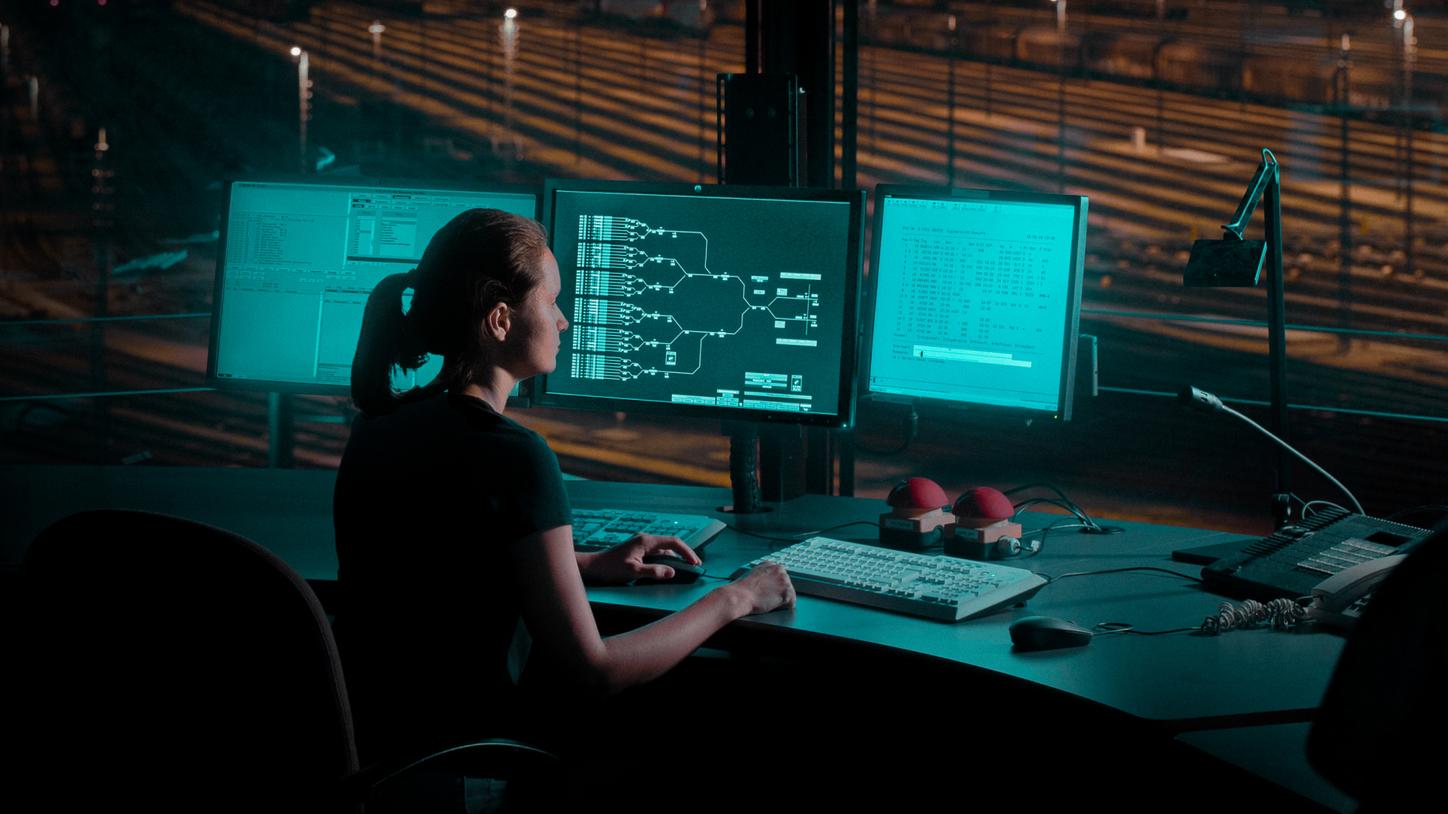
Rail transport is becoming more reliable
Deutsche Bahn, Germany’s national rail operator, sees the virtual representation of trains as the key to achieving greater vehicle reliability. Artificial intelligence is used to simulate vehicle functions – and the real trains are then sent to the workshop precisely when it becomes necessary.
A digital twin for everyone?
In other words, digital twin technology can already improve many aspects of industrial processes, but it’s much more difficult to put the resulting findings to use in medical applications. Creating a general representation of a patient would require neural networks to be trained using millions of datasets. Only then could this data be assembled into a holistic, human model to draw conclusions for a specific patient by comparing their individual initial status with similar data sets.
Today, decisions regarding treatment options and drug administration call for a great deal of flexibility and often still come down to trial and error. After all, the success or failure of a course of treatment can be influenced by a patient’s age, sex, or genetic predispositions, as well as by the complex biochemical processes taking place inside the body.
Neural networks
Personalized medicine is gaining ground
Rather than blanket statements about likely reactions in specific age groups or life situations, this would take the form of an individualized prediction of the side effects of drugs, for example. Alternatively, it could allow treatment decisions to be made based on a specific patient’s status – since patient reactions could be predicted in advance and at no risk. Nevertheless, the technology is still a long way from delivering a complete, lifelong, physiological model of a patient that is updated with every clinical image, every measured blood value, and every completed examination.
That being said, digital twins of individual parts of the body are already within reach. These differ from conventional 3D models because of their highly dynamic nature and the multitude of scenarios that they can be used to run through. Organ models simulate the structure and mode of operation of an organ or organ system, while disease models reveal all of the pathological processes observed as part of a developing disease. In the future, digital twins could also have applications in hospital management.
Reducing risks and saving money
Facilitating treatment decisions and relieving the burden on medical teams
What is a cohort analysis?
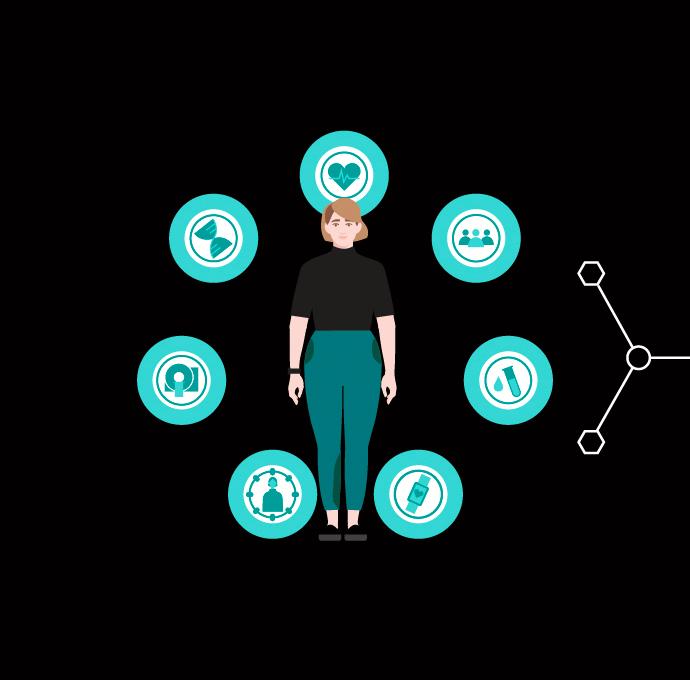
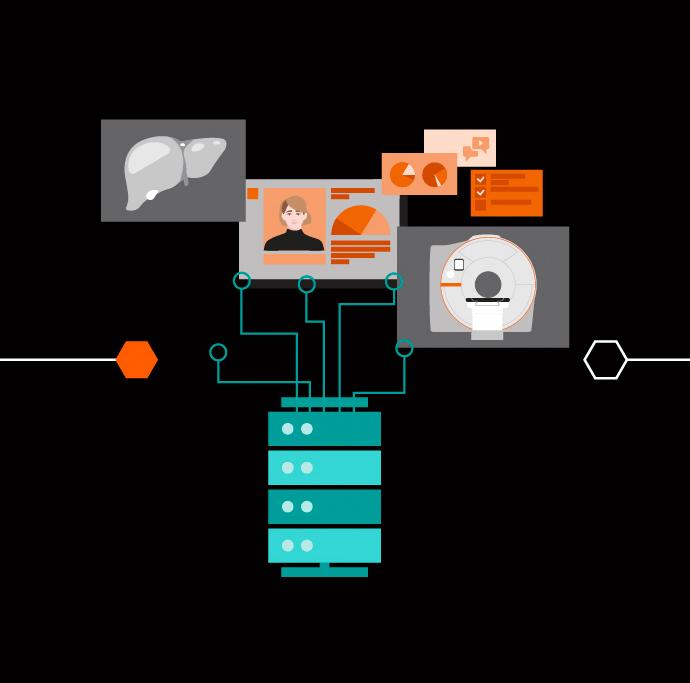
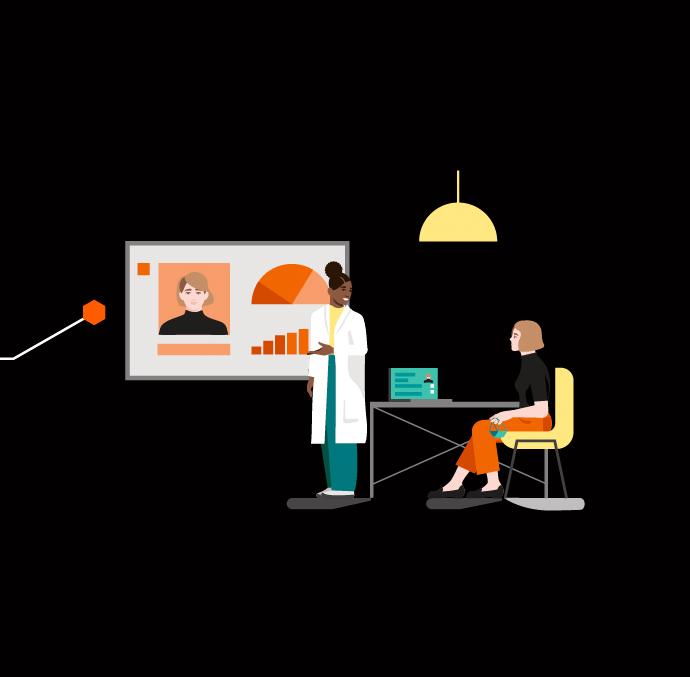
For example, there is a family of AI-powered, cloud-based workflow solutions that can help physicians to reduce the burden of basic repetitive tasks and improve their diagnostic precision when interpreting medical images.
AI-Rad Companion incorporates algorithms that enable the automatic post-processing of image datasets. Meanwhile, the applications of Pathway Companion support personalized and standardized patient management while also providing valuable insights in order to drive process optimization. This leaves radiologists to focus on important tasks in the face of increasing demands.
What is holding the twins back?
One day, AI will be able to advise us about our future health on an individual basis. Does that mean that modern medical practice, which is still used to treat diseases today, will soon be superseded by a form of medicine that keeps watch over our health instead? Will diseases take a less severe course in the future because we can detect them earlier and treat them more accurately? It’s possible – but there are more than just the technical challenges to overcome beforehand.
Although every modern healthcare company maintains its own data infrastructure, these resources largely remain in their respective data silos. There are ample reasons for not disclosing this data: concerns around data protection, fear of the competition’s hunger for data, and the idiosyncrasies of the technology. That poses a dilemma, however, because this wealth of information would make it easier to reach well-founded therapeutic decisions, reduce side effects, plan hospitals according to the needs of patients, and customize medical devices.
In any case, the creation of any individual virtual doppelganger is fundamentally reliant on the timely availability of a complete set of carefully collected data. What’s more, this data must be maintained and supplemented throughout a person’s life and even after their death – for the benefit of future generations. This is the very crux of the matter: It’s precisely the data that we guard like life itself that is required to create a digital twin in the first place.
Women aged 50 and over are regularly called in for a mammogram – an X-ray of the breast – with the aim of detecting breast cancer at an early stage. Depending on individual circumstances, this screening might be too early, too late, or not necessary at all. “A digital twin allows us to make a better assessment of the situation,” says Professor Michael Uder, deputy medical director of Universitätsklinikum Erlangen. It also provides physicians with a new tool that allows them to tailor treatment precisely to the individual patient, says Uder.5
One key advocate of the development of a digital twin for breast cancer screening is d.hip, an alliance made up of Siemens Healthineers, Friedrich-Alexander-Universität Erlangen-Nürnberg, Universitätsklinikum Erlangen, and Medical Valley. The aim is to give women the option of being accompanied by “digital sisters” in as little as around five years.
Andrea Lutz is a journalist and business trainer specialising in medicine, technology and healthcare IT. She lives in Nuremberg, Germany. Doreen Pfeiffer studied journalism with a focus on medicine/bioscience and works as an editor at Siemens Healthineers.
- 1https://www.cell.com/fulltext/S0092-8674(15)01481-6, November 2015.
2Reinhard Laubenbacher, James P. Sluka, James A. Glazier: Using digital twins in viral infection. Science, 12 Mar 2021: Vol. 371, Issue 6534, pp. 1105-1106.
3Source: Hirsch-Kreinsen; Kubach, U.; Stark, R.; Wichert, G. von; Hornung, S.; Hubrecht, L.; Sedlmeir, J.; Steglich, S.: Themenfelder Industrie 4.0. Forschungs- und Entwicklungsbedarfe zur erfolgreichen Umsetzung von e 4.0 [“Industry 4.0 talking points. Research and development requirements for the successful implementation of e 4.0”]. Munich, 2019.
4Meyer, K.; Ostrenko, O.; Bourantas, G.; Morales-Navarrete, H.; Porat-Shliom, N.; Segovia-Miranda, F.; Nonaka, H.; Ghaemi, A.; Verbavatz, J.-M.; Brusch, L;. Sbalzarini, I.F.; Kalaidzidis, Y.; Weigert, R.; Zerial, M.: A Predictive 3D Multi-Scale Model of Biliary Fluid Dynamics in the Liver Lobule. Cell Systems 22, (2017) 277–290.