Home
Perspectives
- Patient twin: Why Sophia is no longer afraid of cancer
Patient twin: Why Sophia is no longer afraid of cancer
Well, to answer one question with another: Have you heard of the “innovator's dilemma”? Clayton M. Christensen described the concept in his 1997 book “The Innovator's Dilemma: When New Technologies Cause Great Firms to Fail.” He argues that established companies often overlook and miss out on waves of innovation within their industries. While focusing on keeping their existing customers happy and maintaining their market share, they neglect to engage with disruptive innovations, missing out on new technologies and business models that fundamentally change existing markets and industries.
Peter Aulbach is certain that this will not happen at Siemens Healthineers: “The concept of a technology vision is a way of showing the public that we are a company that is not afraid of risks, one that invests extensively in future technology and thinks about designing for the future, and yet always puts people – patients and employees in the healthcare industry – at the heart of everything.”
An engineer with a doctorate in radiology and neurosurgery, Aulbach works in the Innovation Strategy and Ecosystem unit at Siemens Healthineers. This is where technology visions are developed: Future scenarios are created around key technologies for treatment paths in major disease areas from the perspectives of patients and medical professionals. The visions include sensor technology, artificial intelligence, digital twinning and robotics, as well as virtual and augmented reality.
Following the creation of future scenarios that address stroke and cardiovascular disease a company-wide technology vision focused on cancer is now being developed under Aulbach's content leadership and coordination. Under the patronage of Chief Technology Officer Peter Schardt, a core team of more than 60 experts from across the business and across different functions have created scenarios by applying design-thinking methods in interdisciplinary co-creation workshops.
A live sketch artist produced drawings of the various development stages of the scenarios to establish a common understanding and basis for further iterations.
Over 200 specialists including those from technical development, sales, and product management joined key experts for future technologies and selected research cooperation partners to systematically review the content. They focused on aspects such as technical feasibility, customer wishes, marketability, and whether the scenarios matched our company's values. The results then provided recommendations to the departments involved on future research and development roadmaps.Patient twinning in cardiology
John's story helps us to answer questions such as: How can we best use digital twins to integrate AI, robots, and sensors in the field of cardiology?Learn more here
These thought experiments aim to create a shared mission statement for all innovators at Siemens Healthineers, a company that operates in an international, highly specialized, and branched structure. “They guide them toward an end-to-end value stream across all devices and services involved, right through to the patients and our customers,” explains Aulbach. Such initiatives promote networked collaboration across the organizational boundaries of the individual businesses, because it takes years of cross-departmental cooperation to implement complex future concepts such as digital patient twinning.
Some aspects of the technology vision have already become reality in the form of semi-automated processes that are integrated into our medical technology products. This is already making life easier for medical professionals as well as patients. Some aspects are currently being implemented, while others have yet to be created. A complete “patient journey” like the one described below could become a reality in about ten years. We could ease the work of medical staff and optimize clinical processes even more. And, crucially, we could tailor treatments for individual cancer patients to an even greater personalized level, and further improve their life expectancy.
We paint a picture of the future in which our key technologies provide doctors and their patients with what they need to successfully fight cancer.
Peter Aulbach, Head of Siemens Healthineers Innovation Center in Erlangen, Germany
Curious to learn more? Find out how the future of cancer therapy could look thanks to developments in medical technology. Follow the journey of our fictitious patient, Sophia. While our innovation team, of course, considered many different types of cancer and treatment options, only ONE possible scenario is presented here to illustrate how a future product and solution portfolio could improve cancer care.
Sophia has been having a tough time. Two years ago, the 58-year-old was diagnosed with lung cancer – more specifically, with non-small-cell lung cancer (NSCLC). [1] A few months ago, further metastases, so-called oligometastases, formed in her liver and spine – a shock for Sophia and her family.
What is NSCLC?
This oncological term describes a special type of cancer metastasis. Metastases are tumors that have spread from the original tumor's site (which is called the primary tumor) to other parts of the body. Oligometastases are a form of metastasis in which only a limited number of metastases have formed in a limited number of organs or tissue parts. They are a stage between localized and advanced systemic cancer. [2]
Initial treatment of all tumors has proven successful. That's why Sophia is now able to not focus on her illness in everyday life. She feels quite reassured. Her health is monitored through regular check-ups with her medical team of oncologists and radiologists, via special apps on her smartphone, and by modern medical technology – all with the help of artificial intelligence, of course.
All medical data collected over the course of Sophia's treatments flow into the digital model of the patient: her digital patient twin. AI continuously evaluates her data in real time so that it can make predictions about the development of Sophia's health. Large language models help to structure disordered data and convert this information into medical reports in language appropriate for Sophia's family doctor, her oncologist, or for Sophia herself.
What are large language models?
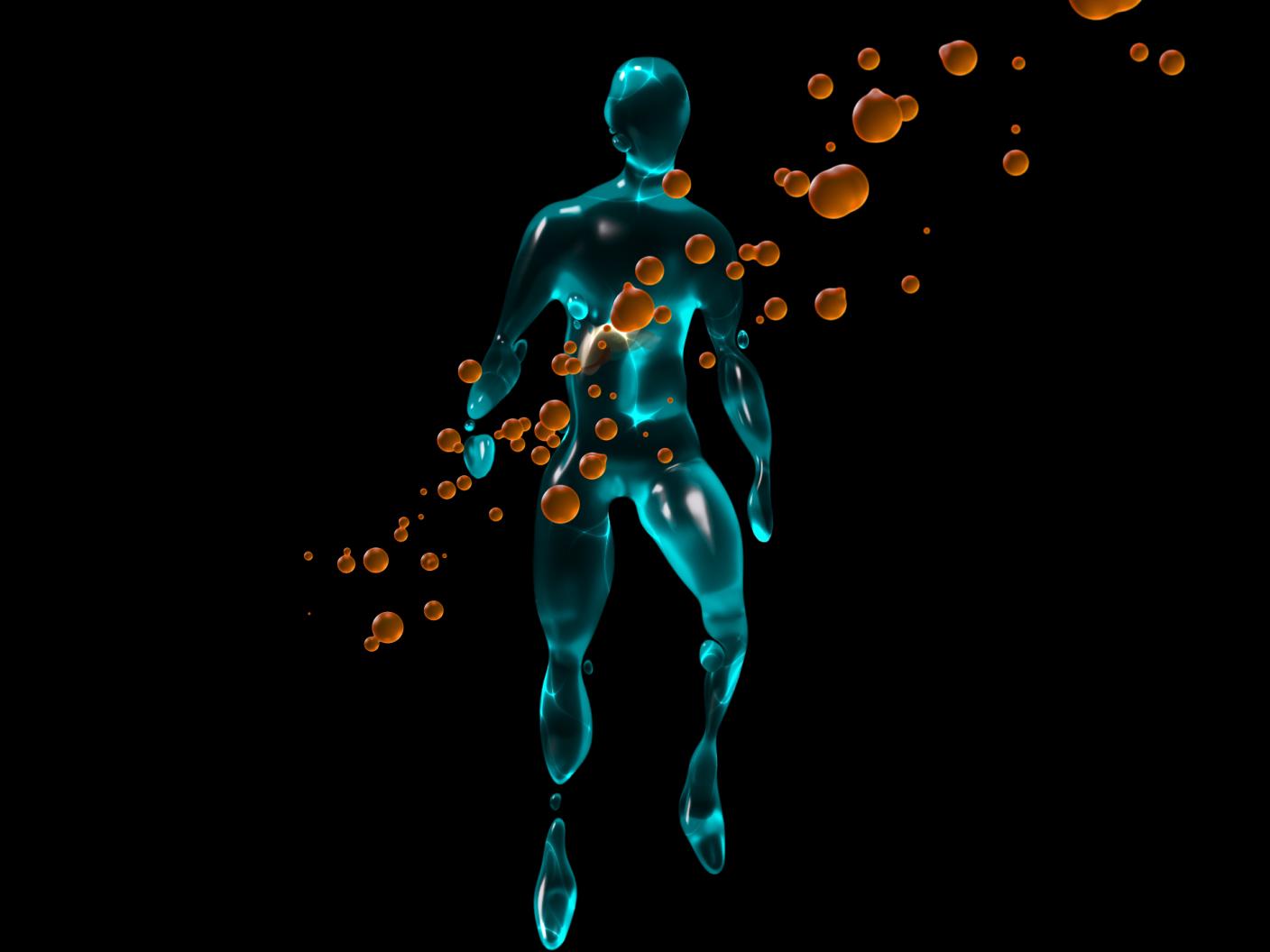
The data are also used in preparing the medical equipment that Sophia needs for her examinations, applying the optimal settings for her individual needs. This not only results in more precise treatment, but also saves time and capacity for medical staff.
Holistic digital support
The digital patient model knows the course of Sophia's treatment and the onward plan that she has agreed with her doctors. It knows Sophia's personal preferences and family situation. Via an app on her smartphone, the digital model reminds Sophia when an examination or treatment is coming up. Based on scientific recommendations, her digital twin offers tips and tricks on what she can do before, during, and after a particular treatment to enhance her general condition and well-being.
The data from Sophia's digital patient twin not only help Sophia herself; she has agreed to donate her data ─ in anonymized form, of course. The data is fed into a giant database, that can be used to train AI algorithms, allowing the AI-based decision-making to be constantly improved.
How are huge amounts of data managed?
A digital patient twin¹ is still a future dream, but Siemens Healthineers is already working to make it a reality. The concept involves creating a digital patient twin based on a person's medical history and their electronic health record (EHR). This information is then updated with data collected from medical examinations and sensors placed, for example on wearable devices such as smart watches. Medical and lifestyle-related data (such as on nutrition) are provided in real time. AI algorithms continuously compare these data with comparable datasets. Simulations are created, for instance to show the person’s expected state of health during treatment. They help to structure the flood of data and enable communication between various devices and systems. All this information provides a basis for supporting patients and medical professionals in their decision-making processes.
Artificial intelligence helps medical centers simulate therapeutic successes so that the best next step can be chosen. Early warnings based on the data collected allow medical centers not only to schedule appointments for check-ups, diagnostics and follow-up treatment, but also to predict bottlenecks in therapy capacity and adjust their human resources accordingly.
1. Screening and early detection
Once a week, Sophie performs a liquid biopsy in the morning after brushing her teeth, a test aimed at detecting cancer cells in the blood. She draws the blood sample herself by simply pricking her finger.
Blood test based on biosensor technology
A liquid biopsy is an examination procedure in which blood samples are used to obtain information about a cancer case. The liquid biopsy technique can detect tumor cells or tumor DNA in the blood. While conventional biopsies involve taking tissue samples, liquid biopsy is a noninvasive procedure that is more comfortable for patients and entails fewer risks. What's more, in some cases it can detect cancer at an earlier stage than conventional biopsies. [3]
Today, an alarm has sounded in Sophia's portable biosensor device: the biosensors have detected abnormalities in her blood counts that could indicate that her cancer has progressed. At the same time, the AI sends a warning message to Sophia's medical team and suggests a strategy for further action. Sophia is worried.
The lead oncologist calls Sophia directly and reassures her. Following the AI's recommendation, she decides together with Sophia that the next diagnostic step should be a computed tomography (CT) scan. The AI in the patient twinning app on Sophia's smartphone identifies the best CT device for her examination. The AI checks available resources and arranges an appointment with Sophia for the CT scan, including the exact location and travel options. Sophia is even told who will meet her to carry out the CT scan.
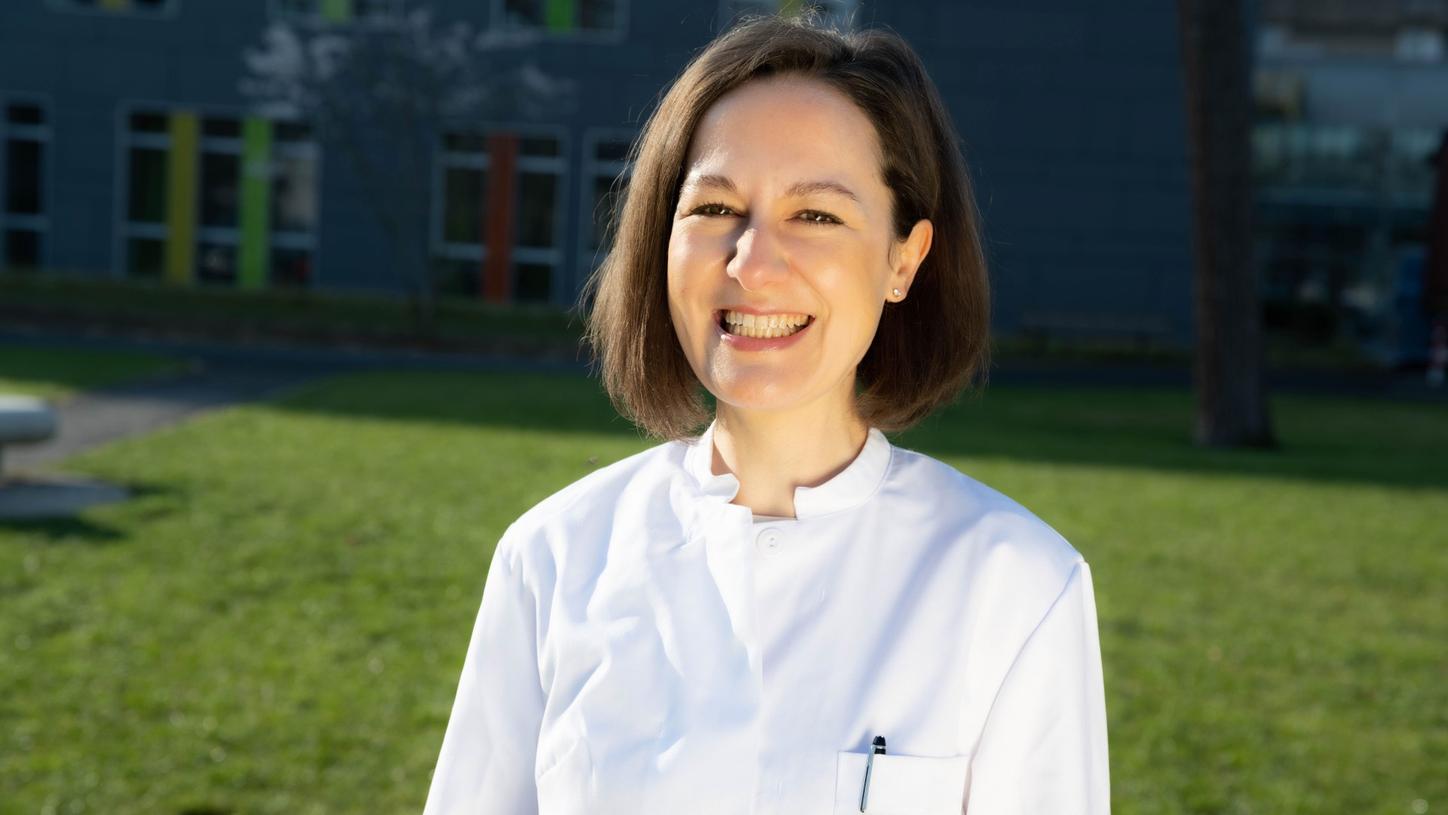
What do physicians think about digital twinning in cancer therapy?
“Digital twins will be essential to make precision medicine a reality,” says Ulrike Attenberger. Here you can read an interview with Professor Attenberger, who heads the Department of Radiology at Bonn University Hospital.
Two days later, Sophia makes her way to her CT scan. Based on the data provided by the patient twin, which has already stored the updated data from Sophia's blood test, the scanner unit is optimally adjusted to her specific needs – even before she enters the medical facility.
The radiology technologist onsite simply has to check and confirm the automatically adjusted settings on the equipment. Radiology technologists could even do this from their computer at home, or while on the go using a tablet. State-of-the-art technology makes it possible to carry out complex medical examinations at any time, even in remote areas or regions where medical staff are in short supply.
Working from home as a medical radiology technologist?
Photon-counting CT scanner
The CT scanner that the AI recommended for Sophia performs photon-counting computed tomography. Its advantages include a reduced radiation dose and improved image quality. [4] The scanner's particularly high-resolution and high-contrast images help medical staff to recognize small details and differences in tissues, and thus delineate the tumor invasion. In combination with AI, radiomics allows further key information about the tumor to be obtained from the data.
Redefining computed tomography
Radiomics is a method used in medical image processing. Large amounts of quantitative data not readily visible to the naked eye (e.g., on the size, shape or texture of a tumor) are extracted from medical images.
The data generated from the images are analyzed with the help of computer programs and algorithms. The information obtained in this way can help to better understand the stage of the disease, make predictions about the progression of the disease, and plan the most appropriate treatment.
Radiomics is still a relatively new area of research, but it has the potential to fundamentally change cancer treatment, detect cancers earlier, and improve the effectiveness of treatment through personalized therapy. [5]
2. Diagnosis and treatment decision-making
A team of medical specialists looks after Sophia, with oncologists from various disciplines working together from different locations. They meet virtually in an online tumor board – a kind of digital round table. The aim is to make a reliable diagnosis for Sophia based on all the data collected so far from her digital patient twin, and to evaluate further treatment options.
All the information is presented visually in the form of a patient avatar. Virtual reality (VR) and augmented reality (AR) headsets help medical staff better understand and interact with the multi-layered information.
Unfortunately, the initial suspicion is confirmed. By comparing images from Sophia's earlier CT and magnetic resonance imaging (MRI) scans as well as scans from a large pool of data from other patients, the photon-counting CT working in tandem with AI identifies patterns that the human eye cannot yet detect: a new tumor is growing in Sophia's left lung.
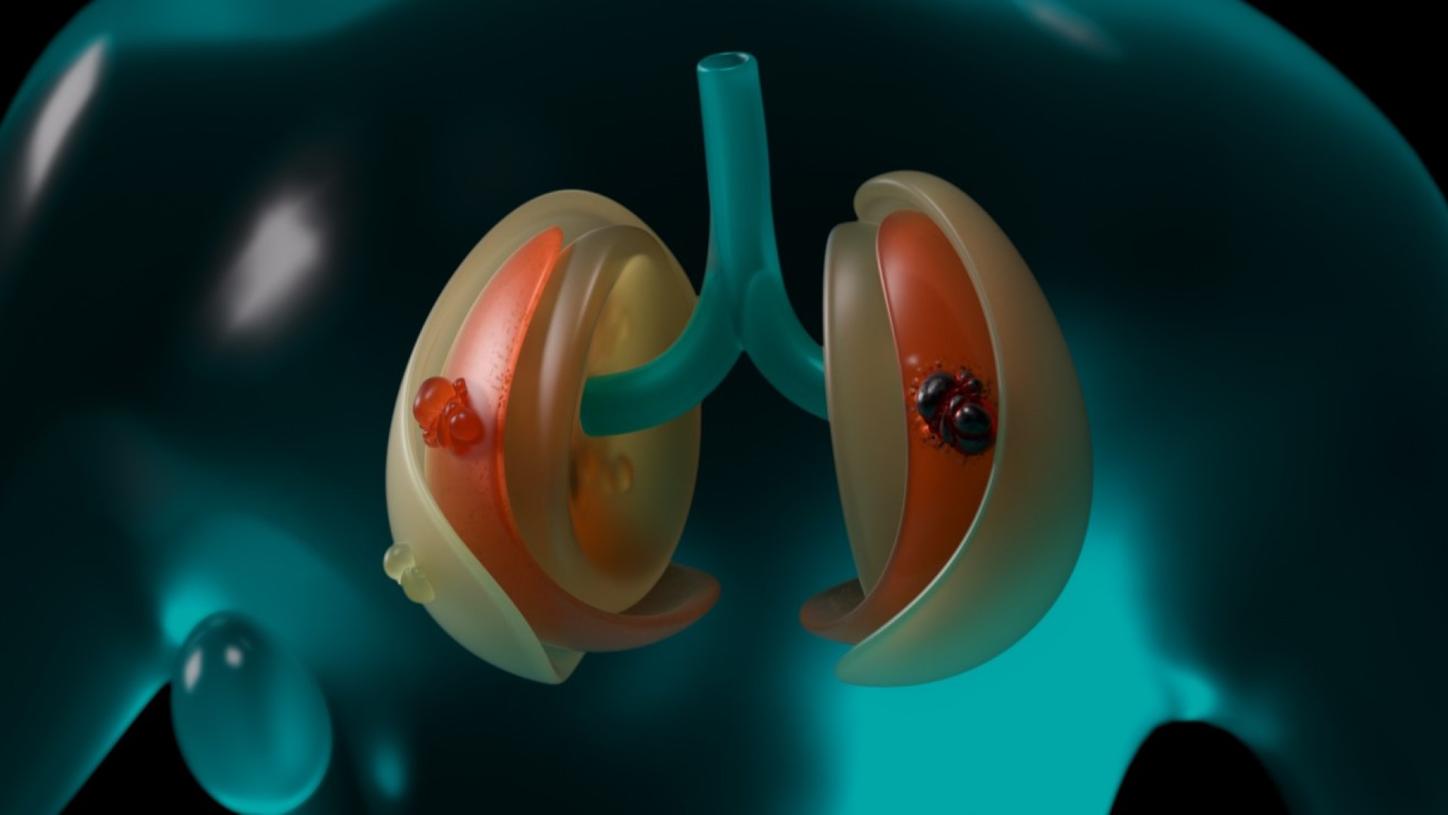
Extended reality (XR) in medical technology?
Fortunately, the tumor is still at a very early stage. The avatar shows exactly where it is located. On request, the physicians also receive further data, for example about Sophia's vital signs, laboratory tests, and genomics. All this information is automatically stored in Sophia's electronic health record (EHR).
What is an electronic health record (EHR)?
The AI uses the avatar to present possible therapeutic approaches so that the medical team can quickly decide on further treatment: among other things, the AI has created a digital twin of the new tumor based on genetic patterns in complex calculations involving radiomics.
This procedure reduces the number of tissue samples required to be taken from the patient. Therapy can be provided in a faster, more targeted manner. Based on the digital twin of the tumor and patient, the AI predicts how likely it is that the tumor will respond to the various forms of therapy and with what success. Can cryoablation [6] or radiation therapy currently better help Sophia?
What is cryoablation?
The AI makes therapy recommendations
Based on the calculated probabilities and taking into account the expected side effects as well as cost efficiency, the AI provides the medical team with therapy recommendations.
Once the physicians have agreed a course of action, Sophia will be brought into the conference. With the help of a large language model, the AI has prepared the complex medical information in a way that is easy for the patient to understand. The doctors inform Sophia about the next recommended treatment steps and the related risks. This gives Sophia clarity and reduces her uncertainty. Sophia ultimately opts to undergo AI-assisted radiation therapy.
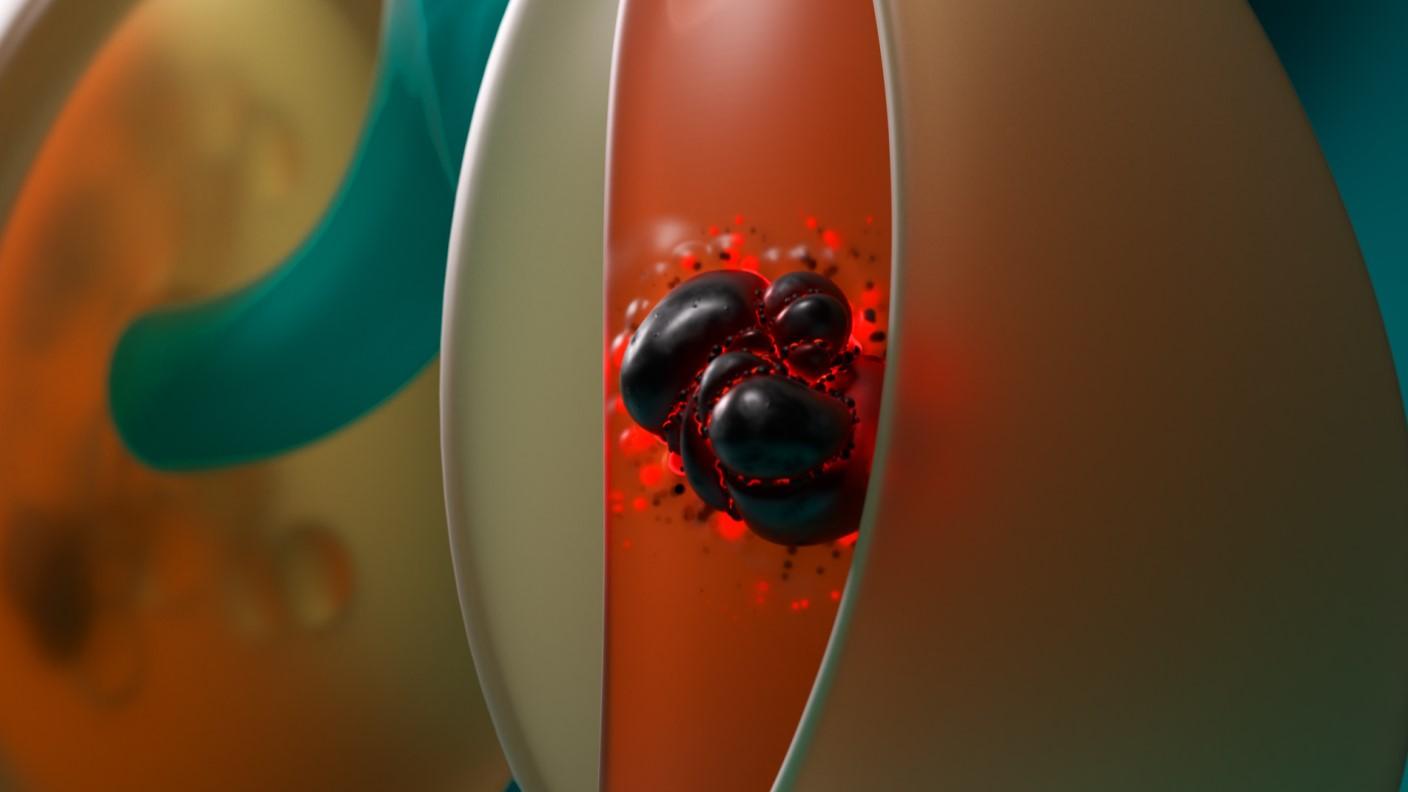
3. Treatment planning and delivery
Before AI-optimized workflows were introduced, radiation therapy planning was a lengthy process that often left patients waiting several weeks. With the support of AI, treatment planning and Sophia's radiation therapy can now be done within a few hours on the same day, so no precious time is lost.
The AI plans capacity, coordinates appointments, assists with the detailed planning of radiation therapy, and adjusts the settings of all devices precisely to Sophia's needs based on her patient data.
The future of radiation therapy
A digital twin for radiation therapy
The AI then generates a digital twin specifically for the planned radiation therapy. It uses the data from Sophia's avatar, such as data from the current CT and MRI scans as well as from the AI-supported recognition (autocontouring) of the organs at risk that need to be protected during irradiation. Determining the boundaries between tumorous and healthy tissue as precisely as possible is critical in the subsequent success of the treatment.
Based on precise data, the AI calculates the amount and distribution of the radiation dose. The radiation oncology specialist checks all the results generated by the AI before the actual treatment starts.
How does autocontouring work?
The AI has calculated that Sophia should receive a total radiation dose of 25 fractions, spread over several weeks. Her first radiation treatment will start immediately. Based on the treatment protocol data, the linear accelerator (linac) settings have already been adjusted to deliver exactly the calculated dose.
The linac has a built-in imaging function that generates more medical images during treatment. It recognizes how the patient's soft tissues and organs move so that it can compensate for these movements. The sensor technology and AI integrated in the linac take into account Sophia's breathing and breathing pauses during irradiation. With the help of this imaging and AI, the radiation dose can be precisely concentrated on the tumor while sparing the surrounding tissue. During quality control, physicians can see whether the treatment was successful, even while it is still ongoing.
What is a linear accelerator, or linac?
Quick adjustment of treatments
For the second radiation dose a few days later, the AI has already evaluated the digital twin for radiation therapy for the results of the first fraction. It recommends an adjustment of the treatment's prescribed dose. The radiation oncologist then decides whether to accept the proposed adjustment to the radiation dose for the second fraction. After consulting with Sophia, he approves the protocol.
Sophia's liver tumor needed treatment about a year ago. At that time, her doctors selected a transcatheter arterial chemoembolization (TACE) on the advice of the AI.
The transcatheter arterial chemoembolization (TACE) procedure combines the principles of embolization and chemotherapy. TACE is often used in the treatment of liver cancer. During an angiography procedure, the specialist in interventional radiology injects small plastic particles into the artery supplying blood to the tumor. The vessel becomes blocked, and the blood supply to the tumor is stopped. The lack of nutrients and oxygen causes the tumor cells in this area to die. In addition, a chemotherapeutic agent can be injected through the catheter, which also results in the death of cancer cells. This can slow down tumor growth. [7]
In conjunction with other key technologies, the AI has also supported TACE, firstly by recommending to the medical team the appropriate guidewires and catheters to use as well as the best embolization material for targeted vascular occlusion. The AI simultaneously checked whether that material was available in the hospital's inventory. For the angiography to embolize the blood supply to the tumor, the AI recommended the correct injection site, access routes, and the best angle for the C-Arm.
Even further in the future, patients like Sophia could perhaps be helped by a surgical angio robot in a specialized medical center. Under constant control and monitoring by the medical professionals, who can intervene remotely at any time, the surgical angio robot could automatically guide the catheter through the blood vessels and inject the necessary drugs as well as materials for the vascular occlusion of the tumor. This would allow difficult, rarely performed and time-critical procedures to be offered in remote, rural areas as well.
What is a C-arm?
Cancer remains a major burden
died of cancer worldwide in 2020. Almost one in six of all deaths was due to cancer.
of delay in treating cancer can lead to increased mortality in seven common types of cancer.
of lung cancer patients are already in stage III or IV at diagnosis due to inadequate early detection.
cancer deaths could be prevented if patients in low- and middle-income countries received better care.
Today, the success of a medical intervention depends largely on the dexterity and experience of the surgical team. Robots, or so-called “co-bots” (collaborative robots that work together with humans), could in future provide greater standardization and precision to deliver treatment of equal quality for all patients. Robots are already being used in minimally invasive operations. They can save doctors who have to perform radiological examinations from exposure to radiation. In some cases, they are already enabling medical experts, who control them from hundreds of kilometers away, to perform remote procedures regardless of location, or assisting in the operating room as mobile service robots by handing sterile materials such as stents, catheters, and swabs to the surgical team. In short, robots could make complex interventions possible in environments that lack the necessary general conditions, for example due to the remoteness of a location or an overload or shortage of skilled medical professionals.
If Sophia needs a minimally invasive surgical procedure, the physicians could use virtual reality to stage and simulate the procedure in advance. In this way, they could save valuable time in the operating theater and minimize the risk of complications during the procedure. In the operating theater itself, augmented reality headsets would be used so that doctors have all the important information about Sophia's health literally “in view” at all times.
The information in their headsets would navigate the surgeons through the procedure. Experts who cannot be onsite could be linked in via the headset to give their assessment. During the procedure, AI would provide real-time support with decision-making. In this way, the process and progress of the operation could be simulated and recommendations or warnings could be given at an early stage if the AI detects deviations from the planned procedure or an impending complication.
4. Medical aftercare
How is Sophia doing a few weeks later? Her radiation therapy has been completed. The current evaluations of her avatar confirm that the treatment has successfully combated the tumor in her lung.
Sophia's life goes on. But her cancer is a chronic disease that she herself and her doctors are monitoring closely: Sophia regularly shares her health status with her treatment team via the app on her smartphone, reliably attends her follow-up examinations based on reminders in the app, and takes her weekly blood test at home.
An app for increasing patient participation?
Sophia feels well cared for: If even the slightest sign of a deterioration in her condition should appear, her digital twin would immediately warn her and the doctors treating her. The AI, together with her medical team, would then find an evidence-based, personalized treatment option for Sophia – and continue to help her manage her health.
We need more than just innovative technological developments and visions if digital patient twins and intelligently networked key technologies are to one day become reality: It will take reliable digital infrastructure, for example, that enables a continuous exchange of medical data. The foundations for this would need to be established in today's healthcare systems and hospitals so that patients and medical staff can benefit from them in the future.
Share this page
Disclaimer:
This text describes future ideas and concepts. It is not intended to describe specific performance and/or safety characteristics of currently planned or future products. Future realization and availability cannot be guaranteed.
¹ Patient twinning is currently under development. It is not for sale. Its future availability cannot be guaranteed.
Sources:
[1] https://www.esmo.org/content/download/123909/2350201/file/DE-Nicht-Kleinzelliges-Bronchialkarzinom-(NSCLC)-Patientenleitlinie.pdf
[2] https://healthcare-in-europe.com/de/news/mittendrin-statt-nur-dabei.html#:~:text=Der%20Begriff%20%E2%80%9EOligometastasierung%E2%80%9C%2C%20der,bei%20ihnen%20nur%20vereinzelte%20(griech.
[3] https://flexikon.doccheck.com/de/Liquid_Biopsy#:~:text=Liquid%20Biopsy%20erm%C3%B6glicht%20den%20Nachweis,den%20Patienten%20zu%20erreichen%20sind.
[4] https://link.springer.com/article/10.1007/s00117-021-00812-8
[5] https://www.drg.de/de-DE/3608/whitepaper/
[6] https://www.mayoclinic.org/tests-procedures/cryoablation-for-cancer/about/pac-20385216
[7] https://www.krebsgesellschaft.de/onko-internetportal/basis-informationen-krebs/krebsarten/andere-krebsarten/leberkrebs/therapie.html